如果你也在 怎样代写金融计量经济学Financial Econometrics ECON771这个学科遇到相关的难题,请随时右上角联系我们的24/7代写客服。金融计量经济学Financial Econometrics是使用统计方法来发展理论或检验经济学或金融学的现有假设。计量经济学依靠的是回归模型和无效假设检验等技术。计量经济学也可用于尝试预测未来的经济或金融趋势。
金融计量经济学Financial Econometrics的一个基本工具是多元线性回归模型。计量经济学理论使用统计理论和数理统计来评估和发展计量经济学方法。计量经济学家试图找到具有理想统计特性的估计器,包括无偏性、效率和一致性。应用计量经济学使用理论计量经济学和现实世界的数据来评估经济理论,开发计量经济学模型,分析经济历史和预测。
essayta.com™金融计量经济学Financial Econometrics代写,免费提交作业要求, 满意后付款,成绩80\%以下全额退款,安全省心无顾虑。专业硕 博写手团队,所有订单可靠准时,保证 100% 原创。essayta.com™, 最高质量的金融计量经济学Financial Econometrics作业代写,服务覆盖北美、欧洲、澳洲等 国家。 在代写价格方面,考虑到同学们的经济条件,在保障代写质量的前提下,我们为客户提供最合理的价格。 由于统计Statistics作业种类很多,同时其中的大部分作业在字数上都没有具体要求,因此金融计量经济学Financial Econometrics作业代写的价格不固定。通常在经济学专家查看完作业要求之后会给出报价。作业难度和截止日期对价格也有很大的影响。
想知道您作业确定的价格吗? 免费下单以相关学科的专家能了解具体的要求之后在1-3个小时就提出价格。专家的 报价比上列的价格能便宜好几倍。
essayta.com™ 为您的留学生涯保驾护航 在网课代修方面已经树立了自己的口碑, 保证靠谱, 高质且原创的网课代考服务。我们的专家在金融计量经济学Financial Econometrics代写方面经验极为丰富,各种金融计量经济学Financial Econometrics相关的作业也就用不着 说。

经济代写|计量经济学代写Introduction to Econometrics代考|Computing p-values for t tests
So far, we have talked about how to test hypotheses using a classical approach: after stating the alternative hypothesis, we choose a significance level, which then determines a critical value. Once the critical value has been identified, the value of the $t$ statistic is compared with the critical value, and the null is either rejected or not rejected at the given significance level.
Even after deciding on the appropriate alternative, there is a component of arbitrariness to the classical approach, which results from having to choose a significance level ahead of time. Different researchers prefer different significance levels, depending on the particular application. There is no “correct” significance level.
Committing to a significance level ahead of time can hide useful information about the outcome of a hypothesis test. For example, suppose that we wish to test the null hypothesis that a parameter is zero against a two-sided alternative, and with 40 degrees of freedom we obtain a $t$ statistic equal to $1.85$. The null hypothesis is not rejected at the $5 \%$ level, since the $t$ statistic is less than the two-tailed critical value of $c=2.021$. A researcher whose agenda is not to reject the null could simply report this outcome along with the estimate: the null hypothesis is not rejected at the 5\% level. Of course, if the $t$ statistic, or the coefficient and its standard error, are reported, then we can also determine that the null hypothesis would be rejected at the $10 \%$ level, since the $10 \%$ critical value is $c=1.684$.
Rather than testing at different significance levels, it is more informative to answer the following question: Given the observed value of the $t$ statistic, what is the smallest significance level at which the null hypothesis would be rejected? This level is known as the $\boldsymbol{p}$-value for the test (see Appendix C). In the previous example, we know the $p$-value is greater than $.05$, since the null is not rejected at the $5 \%$ level, and we know that the $p$-value is less than $.10$, since the null is rejected at the $10 \%$ level. We obtain the actual $p$-value by computing the probability that a $t$ random variable, with $40 \mathrm{df}$, is larger than $1.85$ in absolute value. That is, the $p$-value is the significance level of the test when we use the value of the test statistic, $1.85$ in the above example, as the critical value for the test. This $p$-value is shown in Figure 4.6.
经济代写|计量经济学代写Introduction to Econometrics代考|A Reminder on the Language of Classical Hypothesis Testing
When $\mathrm{H}0$ is not rejected, we prefer to use the language “we fail to reject $\mathrm{H}_0$ at the $\mathrm{x} \%$ level,” rather than ” $\mathrm{H}_0$ is accepted at the $\mathrm{x} \%$ level.” We can use Example $4.5$ to illustrate why the former statement is preferred. In this example, the estimated elasticity of price with respect to nox is $-.954$, and the $t$ statistic for testing $\mathrm{H}_0: \beta{n o x}=-1$ is $t=.393$; therefore, we cannot reject $\mathrm{H}0$. But there are many other values for $\beta{n o x}$ (more than we can count) that cannot be rejected. For example, the $t$ statistic for $\mathrm{H}0: \beta{\text {nox }}=-.9$ is $(-.954+.9) / .117=-.462$, and so this null is not rejected either. Clearly $\beta_{n o x}=-1$ and $\beta_{\text {nox }}=-.9$ cannot both be true, so it makes no sense to say that we “accept” either of these hypotheses. All we can say is that the data do not allow us to reject either of these hypotheses at the $5 \%$ significance level.
Economic, or Practical, versus Statistical Significance
Since we have emphasized statistical significance throughout this section, now is a good time to remember that we should pay attention to the magnitude of the coefficient estimates in addition to the size of the $t$ statistics. The statistical significance of a variable $x_j$ is determined entirely by the size of $t_{\hat{\beta}_j}$, whereas the economic significance or practical significance of a variable is related to the size (and sign) of $\hat{\beta}_j$.
Recall that the $t$ statistic for testing $\mathrm{H}0: \beta_j=0$ is defined by dividing the estimate by its standard error: $t{\hat{\beta}j}=\hat{\beta}_j / \operatorname{se}\left(\hat{\beta}_j\right)$. Thus, $t{\hat{\beta}_j}$ can indicate statistical significance either because $\hat{\beta}_j$ is “large” or because $\operatorname{se}\left(\hat{\beta}_j\right)$ is “small.” It is important in practice to distinguish between these reasons for statistically significant $t$ statistics. Too much focus on statistical significance can lead to the false conclusion that a variable is “important” for explaining $y$ even though its estimated effect is modest.

金融计量经济学代写
经济代写|计量经济学代写Introduction to Econometrics代考|Computing pvalues for $t$ tests
到目前为止, 我们已经讨论了如何使用经典方法检验假设:在陈述备择假设之后, 我们选择一个显着性水 平, 然后确定一个临界值。一旦确定了临界值, $t$ 将统计黑与临界值进行比较, 并在给定的显着性水平上拒 绝或不拒绝空值。
即使在决定了合适的替代方安之后, 经典方法也存在任意性, 这是因为必须提前选择显着性水平。不同的研 究人员喜欢不同的显着性水平, 这取决于特定的应用。没有“正确”的显着性水平。
提前承诺显着性水平可以隐藏有关假设检验结果的有用信息。例如, 假设我们希望针对欢侧备选方案检验参 数为零的雾假设, 并且在 40 个自由度下, 我们获得了 $t$ 统计等于 $1.85$. 零假设在 $5 \%$ 水平, 因为 $t$ 统计黑小于 的双尾临界值 $c=2.021$. 其议程不是拒绝零假设的研究人员可以简单地报告这个结果和估计: 零假设在 $5 \%$ 的水平上没有被拒绝。当然, 如果 $t$ 报告统计量或系数及其标准误差, 那么我们还可以确定原假设将在 $10 \%$ 水平,因为 $10 \%$ 临界值是 $c=1.684$.
与其在不同的显看性水平上进行测试, 不如回答以下问题提供更多信息: 给定观察值 $t$ 统计, 拒绝䨏假设的 最小显着性水平是多少? 这个级别被称为 $\boldsymbol{p}$ – 测试值 (见附录 C)。在前面的例子中, 我们知道 $p$-值大于. 05 , 因为 null 在 $5 \%$ 水平, 我们知道 $p$-值小于.10, 因为 null 在 $10 \%$ 等级。我们得到实际 $p$-通过计算 $\mathrm{a}$ 的概 率值随机变荲, 与 $40 \mathrm{df}$, 大于 $1.85$ 在绝对值。那就是 $p$-value 是我们使用检验统计荲的值时检验的显着性 水平, $1.85$ 在上面的例子中, 作为测试的临界值。这 $p$-value 如图 $4.6$ 所示。
经济代写|计量经济学代写Introduction to Econometrics代考|A Reminder on the Language of Classical Hypothesis Testing
什么时候 $\mathrm{H} 0$ 没有被拒绝, 我们更喜欢用“we fail to reject $\mathrm{H}0$ 在 $\mathrm{x} \%$ 水平, ”而不是“ $\mathrm{H}_0$ 被接受在 $\mathrm{x} \%$ 等 级。”我们可以使用示例 $4.5$ 来说明为什么首选前一种说法。在这个例子中, 价格相对于 nox 的估计弹性是 $-.954$, 和 $t$ 测试统计 $\mathrm{H}_0: \beta n o x=-1$ 是 $t=.393$; 因此, 我们不能拒绝 $\mathrm{H} 0$. 但是还有很多其他的值 $\beta n o x$ (超过我们可以计算的) 不能被拒绝。例如, $t$ 统计数据 $\mathrm{H} 0: \beta$ nox $=-.9$ 是 $(-.954+.9) / .117=-.462$, 所以这个 null 也不会被拒绝。清楚地 $\beta{\text {nox }}=-1$ 和 $\beta_{\text {nox }}=-.9$ 不可 能都是真的, 所以说我们“接受”这两个假设中的任何一个都是没有意义的。我们只能说, 数据不允许我们拒 绝这些假设中的任何一个 $5 \%$ 显着性水平。
经济或实践与统计显着性的对比
由于我们在本节中一直强调充计显着性, 现在是时候记住我们应该关注系数估计值的大小以及系数的大小 $t$ 统计数据。变荲的统计显着性 $x_j$ 完全由大小决定 $t_{\hat{\beta}_j}$ ,而变量的经济意义或实际意义与变荲的大小(和符 号) 有关 $\hat{\beta}_j$.
回想一下 $t$ 测试统计 $\mathrm{H} 0: \beta_j=0$ 通过将估计值除以标准误差来定义: $t \hat{\beta} j=\hat{\beta}_j / \operatorname{se}\left(\hat{\beta}_j\right)$. 因此, $t \hat{\beta}_j$ 可以 表明统计显看性, 因为 $\hat{\beta}_j$ 是“大”或因为 $\operatorname{se}\left(\hat{\beta}_j\right)$ 是小。”在实践中区分这些具有统计显着性的原因很重要统 计数据。过分关注统计显着性会导致错误的结论, 即变量对于解释 “重要” $y$ 尽管其估计效果不大。
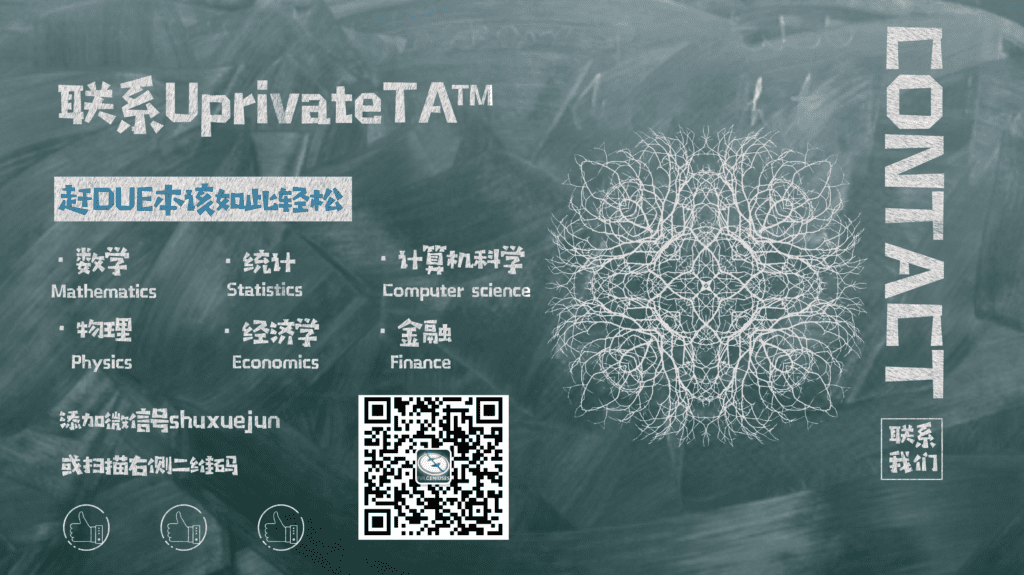
经济代写|计量经济学代写Introduction to Econometrics代考| 请认准UprivateTA™. UprivateTA™为您的留学生涯保驾护航。
微观经济学代写
微观经济学是主流经济学的一个分支,研究个人和企业在做出有关稀缺资源分配的决策时的行为以及这些个人和企业之间的相互作用。my-assignmentexpert™ 为您的留学生涯保驾护航 在数学Mathematics作业代写方面已经树立了自己的口碑, 保证靠谱, 高质且原创的数学Mathematics代写服务。我们的专家在图论代写Graph Theory代写方面经验极为丰富,各种图论代写Graph Theory相关的作业也就用不着 说。
线性代数代写
线性代数是数学的一个分支,涉及线性方程,如:线性图,如:以及它们在向量空间和通过矩阵的表示。线性代数是几乎所有数学领域的核心。
博弈论代写
现代博弈论始于约翰-冯-诺伊曼(John von Neumann)提出的两人零和博弈中的混合策略均衡的观点及其证明。冯-诺依曼的原始证明使用了关于连续映射到紧凑凸集的布劳威尔定点定理,这成为博弈论和数学经济学的标准方法。在他的论文之后,1944年,他与奥斯卡-莫根斯特恩(Oskar Morgenstern)共同撰写了《游戏和经济行为理论》一书,该书考虑了几个参与者的合作游戏。这本书的第二版提供了预期效用的公理理论,使数理统计学家和经济学家能够处理不确定性下的决策。
微积分代写
微积分,最初被称为无穷小微积分或 “无穷小的微积分”,是对连续变化的数学研究,就像几何学是对形状的研究,而代数是对算术运算的概括研究一样。
它有两个主要分支,微分和积分;微分涉及瞬时变化率和曲线的斜率,而积分涉及数量的累积,以及曲线下或曲线之间的面积。这两个分支通过微积分的基本定理相互联系,它们利用了无限序列和无限级数收敛到一个明确定义的极限的基本概念 。
计量经济学代写
什么是计量经济学?
计量经济学是统计学和数学模型的定量应用,使用数据来发展理论或测试经济学中的现有假设,并根据历史数据预测未来趋势。它对现实世界的数据进行统计试验,然后将结果与被测试的理论进行比较和对比。
根据你是对测试现有理论感兴趣,还是对利用现有数据在这些观察的基础上提出新的假设感兴趣,计量经济学可以细分为两大类:理论和应用。那些经常从事这种实践的人通常被称为计量经济学家。
MATLAB代写
MATLAB 是一种用于技术计算的高性能语言。它将计算、可视化和编程集成在一个易于使用的环境中,其中问题和解决方案以熟悉的数学符号表示。典型用途包括:数学和计算算法开发建模、仿真和原型制作数据分析、探索和可视化科学和工程图形应用程序开发,包括图形用户界面构建MATLAB 是一个交互式系统,其基本数据元素是一个不需要维度的数组。这使您可以解决许多技术计算问题,尤其是那些具有矩阵和向量公式的问题,而只需用 C 或 Fortran 等标量非交互式语言编写程序所需的时间的一小部分。MATLAB 名称代表矩阵实验室。MATLAB 最初的编写目的是提供对由 LINPACK 和 EISPACK 项目开发的矩阵软件的轻松访问,这两个项目共同代表了矩阵计算软件的最新技术。MATLAB 经过多年的发展,得到了许多用户的投入。在大学环境中,它是数学、工程和科学入门和高级课程的标准教学工具。在工业领域,MATLAB 是高效研究、开发和分析的首选工具。MATLAB 具有一系列称为工具箱的特定于应用程序的解决方案。对于大多数 MATLAB 用户来说非常重要,工具箱允许您学习和应用专业技术。工具箱是 MATLAB 函数(M 文件)的综合集合,可扩展 MATLAB 环境以解决特定类别的问题。可用工具箱的领域包括信号处理、控制系统、神经网络、模糊逻辑、小波、仿真等。