如果你也在 怎样代写计算机网络Computer Networking CIS654这个学科遇到相关的难题,请随时右上角联系我们的24/7代写客服。计算机网络Computer Networking是一组共享位于网络节点上或由网络节点提供的资源的计算机。这些计算机通过数字互连使用共同的通信协议来相互通信。这些互连是由电信网络技术组成的,基于物理有线、光学和无线射频方法,可以安排在各种网络拓扑结构中。
计算机网络Computer Networking的节点可以包括个人计算机、服务器、网络硬件或其他专用或通用的主机。它们由网络地址识别,也可以有主机名。主机名作为节点的记忆性标签,在最初分配后很少改变。网络地址用于通过通信协议(如互联网协议)来定位和识别节点。计算机网络可按许多标准进行分类,包括用于传输信号的传输介质、带宽、组织网络流量的通信协议、网络规模、拓扑结构、流量控制机制和组织意图。
计算机网络Computer Networking代写,免费提交作业要求, 满意后付款,成绩80\%以下全额退款,安全省心无顾虑。专业硕 博写手团队,所有订单可靠准时,保证 100% 原创。最高质量的计算机网络Computer Networking作业代写,服务覆盖北美、欧洲、澳洲等 国家。 在代写价格方面,考虑到同学们的经济条件,在保障代写质量的前提下,我们为客户提供最合理的价格。 由于作业种类很多,同时其中的大部分作业在字数上都没有具体要求,因此计算机网络Computer Networking作业代写的价格不固定。通常在专家查看完作业要求之后会给出报价。作业难度和截止日期对价格也有很大的影响。
海外留学生论文代写;英美Essay代写佼佼者!
EssayTA™有超过2000+名英美本地论文代写导师, 覆盖所有的专业和学科, 每位论文代写导师超过10,000小时的学术Essay代写经验, 并具有Master或PhD以上学位.
EssayTA™在线essay代写、散文、论文代写,3分钟下单,匹配您专业相关写作导师,为您的留学生涯助力!
我们拥有来自全球顶级写手的帮助,我们秉承:责任、能力、时间,为每个留学生提供优质代写服务
论文代写只需三步, 随时查看和管理您的论文进度, 在线与导师直接沟通论文细节, 在线提出修改要求. EssayTA™支持Paypal, Visa Card, Master Card, 虚拟币USDT, 信用卡, 支付宝, 微信支付等所有付款方式.

CS代写|计算机网络代写Computer Networking代考|Measuring Structural Correlations in Graphs
The rise of the Web, social networks, and bioinformatics has presented the scientists with numerous graphs, each consisting of millions of nodes and edges. Hidden in these large datasets are the answers to important questions in networking, sociology, business, and biology. These graphs not only have topological structures but also contain events/activities that occurred on their nodes. For example, an eBay customer could sell or bid on a product; a Facebook user could play a Zynga game with friends; a computer in the Internet could suffer various attacks from hackers. This complex combination raises new research problems in the graph data analysis (Ester et al., 2006), (Moser et al., 2009), (Silva, Meira, and Zaki, 2010).
Among different events taking place in a network, some exhibit strong correlations with the network structure, while others do not. Such structural correlations might shed light on viral influence existing in the corresponding network, which is the key to many research problems in product marketing (Chen, Wang, and Wang, 2010), online advertisement (Bao and Chang, 2010), and recommendation (Kautz, Selman, and Shah, 1997). Figure $2.1$ shows the distribution of three different events over the same graph. We can easily instantiate Figure $2.1$ into different application scenarios. They could be three different products bought by members in a social network, or three different intrusion alerts raised by computers in a computer network. In terms of the products, dark nodes in Figure 2.1a-c represent the members who purchased the products $A, B$ and $C$, respectively. Intuitively, Figure $2.1$ shows that in this network, people who bought product $A$ (or $B$ ) are closer to each other. On the contrary, black nodes for $C$ seem to be randomly distributed. We name this kind of correlation as self structural correlation (SSC) (Guan et al., 2011). In this scenario, the network would be suitable for promoting $A$ and $B$, and we can promote $A$ and $B$ to people who have not bought them. While it is hard to derive deterministic relationships between sales and the network structure, it is possible to study how the sales is correlated to the structure. In fact, one can raise several interesting questions related to the structure and events distributed over the structure:
In regard to the sales of $A$ and $B$, which one is more related to the underlying social network?
Given two networks $G$ and $G^{\prime}$ for the same group of users, for example, their email network and Facebook network, are the sales of $A$ more related to $G$ than $G^{\prime}$ ?
If we have snapshots of network $G$ during different periods, can we measure how product A was dispersed over the network over time? Was it purchased by a small community at the beginning?
CS代写|计算机网络代写Computer Networking代考|Solutions for Measuring Structural Correlations
We develop a novel measure to assess SSC inca graph. The measure aggregates the proximity among nodes on which the same event has occurred, using a proximity function such as hitting time (Lovász, 1993). We propose a modified version of hitting time named decayed hitting time(DHT) to better and faster calculate SSC. We develop an efficient estimation framework, gScore (Graph structural correlation estimation), to quickly calculate correlation scores in large-scale networks. By estimating the deviation from the expected correlation score of a random situation, our method is able to discover the events of nodes that are highly correlated with the graph structure. We demonstrate gScore’s effectiveness by constructing synthetic events on the DBLP coauthor network. We also discover interesting correlated and uncorrelated products discovered from $w w w$.TaoBao.com, the largest online shopping network in China. Scalability of gScore is tested on the Twitter network.
For TESC, we also design a novel measure and then an efficient estimation framework. Specifically, given the occurrences of two events, we choose a sample of “reference nodes” uniformly from the vicinity of all occurrences and compute for each reference node the densities of the two events in its vicinity, respectively. Then, we employ the Kendall’s $\tau$ rank correlation measure (Kendall and Gibbons, 1990) to compute the average concordance of density changes for the two events, over all pairs of reference nodes. Finally, correlation significance can be efficiently assessed by $\tau$ ‘s nice property of being asymptotically normal under the null hypothesis. For efficiently sampling the reference nodes, different sampling techniques are proposed to shorten the statistical testing time. Our framework is scalable to very large graphs. We demonstrate the efficacy of the TESC testing framework by event simulations on the DBLP graph. We further test its scalability in a Twitter graph with 20 million nodes. Case studies of applying the testing framework on real events occurring on real graphs are provided with interesting results.

计算机网络代写
CS代写|计算机网络代写计算机网络代考|测量图中的结构相关性
网络、社交网络和生物信息学的兴起为科学家们提供了无数的图表,每一个都由数百万个节点和边组成。隐藏在这些大型数据集中的是网络、社会学、商业和生物学等领域重要问题的答案。这些图不仅具有拓扑结构,而且还包含在其节点上发生的事件/活动。例如,eBay的客户可以出售或竞标一件产品;Facebook用户可以与好友一起玩Zynga游戏;互联网上的计算机可能遭受黑客的各种攻击。这种复杂的组合在图数据分析中提出了新的研究问题(Ester et al., 2006), (Moser et al., 2009), (Silva, Meira和Zaki, 2010)。在网络中发生的不同事件中,有些与网络结构有很强的相关性,而有些则没有。这种结构相关性可能有助于揭示在相应网络中存在的病毒式影响,这是产品营销(Chen, Wang, and Wang, 2010)、在线广告(Bao and Chang, 2010)和推荐(Kautz, Selman, and Shah, 1997)中许多研究问题的关键。图$2.1$显示了三个不同事件在同一图上的分布。我们可以很容易地在不同的应用程序场景中实例化图$2.1$。它们可能是一个社交网络中的成员购买的三种不同的产品,也可能是由计算机网络中的计算机发出的三种不同的入侵警报。产品方面,图2.1a-c中的暗节点分别代表购买产品$A, B$和$C$的会员。直观地看,图$2.1$显示在这个网络中,购买产品$A$(或$B$)的人彼此之间的距离更近。相反,$C$的黑节点似乎是随机分布的。我们将这种相关称为自结构相关(SSC) (Guan et al., 2011)。在这种情况下,网络适合推广$A$和$B$,我们可以向没有购买的人推广$A$和$B$。虽然很难推导出销售与网络结构之间的确定性关系,但研究销售如何与网络结构相关是可能的。事实上,人们可以提出几个与结构和分布在结构上的事件相关的有趣的问题
关于$A$和$B$的销售,哪一个与底层的社交网络更相关?
对于同一组用户,假设有两个网络$G$和$G^{\prime}$,例如他们的电子邮件网络和Facebook网络,那么$A$的销售与$G$的关系是否大于与$G^{\prime}$的关系 如果我们有网络$G$在不同时期的快照,我们能测量产品A是如何随着时间在网络中分散的吗?它最初是由一个小社区购买的吗?
CS代写|计算机网络代写计算机网络代考|测量结构相关性的解决方案
我们开发了一种新的方法来评估SSC印加图。该度量通过使用诸如命中时间(Lovász, 1993)之类的接近函数来聚合发生相同事件的节点之间的接近性。为了更好、更快地计算SSC,我们提出了一种改进的命中时间衰减命中时间(DHT)。我们开发了一个高效的估计框架gScore(图结构相关估计),用于快速计算大规模网络中的相关分数。通过估计随机情况与预期相关分数的偏差,我们的方法能够发现与图结构高度相关的节点事件。我们通过在DBLP合著者网络上构建合成事件来证明gScore的有效性。我们还从中国最大的在线购物网站$w w w$ .淘宝网上发现有趣的相关和不相关产品。在Twitter网络上测试gScore的可伸缩性
对于TESC,我们还设计了一种新的测量方法,进而建立了一个有效的估计框架。具体来说,给定两个事件的发生次数,我们从所有事件发生的附近均匀地选择一个“参考节点”样本,并为每个参考节点分别计算其附近两个事件的密度。然后,我们使用Kendall的$\tau$秩相关度量(Kendall and Gibbons, 1990)来计算两个事件在所有参考节点对上的密度变化的平均一致性。最后,利用$\tau$在零假设下渐近正态的良好性质,可以有效地评估相关显著性。为了有效地对参考节点进行抽样,提出了不同的抽样技术,以缩短统计检验时间。我们的框架可以扩展到非常大的图形。我们通过在DBLP图上的事件模拟证明了TESC测试框架的有效性。我们进一步在一个包含2000万个节点的Twitter图中测试它的可伸缩性。将测试框架应用于真实图上发生的真实事件的案例研究提供了有趣的结果
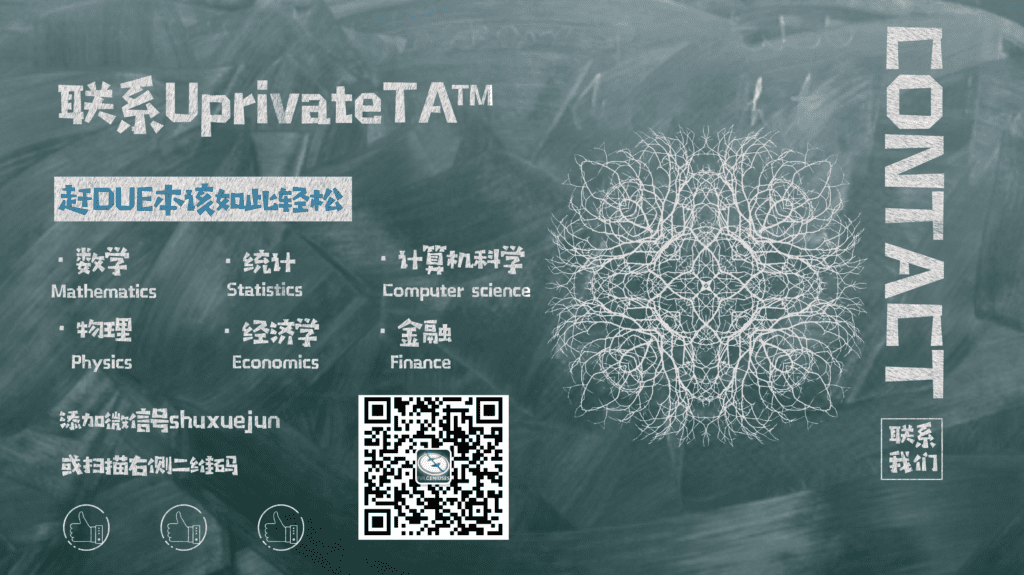
CS代写|计算机网络代写Computer Networking代考 请认准UprivateTA™. UprivateTA™为您的留学生涯保驾护航。
微观经济学代写
微观经济学是主流经济学的一个分支,研究个人和企业在做出有关稀缺资源分配的决策时的行为以及这些个人和企业之间的相互作用。my-assignmentexpert™ 为您的留学生涯保驾护航 在数学Mathematics作业代写方面已经树立了自己的口碑, 保证靠谱, 高质且原创的数学Mathematics代写服务。我们的专家在图论代写Graph Theory代写方面经验极为丰富,各种图论代写Graph Theory相关的作业也就用不着 说。
线性代数代写
线性代数是数学的一个分支,涉及线性方程,如:线性图,如:以及它们在向量空间和通过矩阵的表示。线性代数是几乎所有数学领域的核心。
博弈论代写
现代博弈论始于约翰-冯-诺伊曼(John von Neumann)提出的两人零和博弈中的混合策略均衡的观点及其证明。冯-诺依曼的原始证明使用了关于连续映射到紧凑凸集的布劳威尔定点定理,这成为博弈论和数学经济学的标准方法。在他的论文之后,1944年,他与奥斯卡-莫根斯特恩(Oskar Morgenstern)共同撰写了《游戏和经济行为理论》一书,该书考虑了几个参与者的合作游戏。这本书的第二版提供了预期效用的公理理论,使数理统计学家和经济学家能够处理不确定性下的决策。
微积分代写
微积分,最初被称为无穷小微积分或 “无穷小的微积分”,是对连续变化的数学研究,就像几何学是对形状的研究,而代数是对算术运算的概括研究一样。
它有两个主要分支,微分和积分;微分涉及瞬时变化率和曲线的斜率,而积分涉及数量的累积,以及曲线下或曲线之间的面积。这两个分支通过微积分的基本定理相互联系,它们利用了无限序列和无限级数收敛到一个明确定义的极限的基本概念 。
计量经济学代写
什么是计量经济学?
计量经济学是统计学和数学模型的定量应用,使用数据来发展理论或测试经济学中的现有假设,并根据历史数据预测未来趋势。它对现实世界的数据进行统计试验,然后将结果与被测试的理论进行比较和对比。
根据你是对测试现有理论感兴趣,还是对利用现有数据在这些观察的基础上提出新的假设感兴趣,计量经济学可以细分为两大类:理论和应用。那些经常从事这种实践的人通常被称为计量经济学家。
MATLAB代写
MATLAB 是一种用于技术计算的高性能语言。它将计算、可视化和编程集成在一个易于使用的环境中,其中问题和解决方案以熟悉的数学符号表示。典型用途包括:数学和计算算法开发建模、仿真和原型制作数据分析、探索和可视化科学和工程图形应用程序开发,包括图形用户界面构建MATLAB 是一个交互式系统,其基本数据元素是一个不需要维度的数组。这使您可以解决许多技术计算问题,尤其是那些具有矩阵和向量公式的问题,而只需用 C 或 Fortran 等标量非交互式语言编写程序所需的时间的一小部分。MATLAB 名称代表矩阵实验室。MATLAB 最初的编写目的是提供对由 LINPACK 和 EISPACK 项目开发的矩阵软件的轻松访问,这两个项目共同代表了矩阵计算软件的最新技术。MATLAB 经过多年的发展,得到了许多用户的投入。在大学环境中,它是数学、工程和科学入门和高级课程的标准教学工具。在工业领域,MATLAB 是高效研究、开发和分析的首选工具。MATLAB 具有一系列称为工具箱的特定于应用程序的解决方案。对于大多数 MATLAB 用户来说非常重要,工具箱允许您学习和应用专业技术。工具箱是 MATLAB 函数(M 文件)的综合集合,可扩展 MATLAB 环境以解决特定类别的问题。可用工具箱的领域包括信号处理、控制系统、神经网络、模糊逻辑、小波、仿真等。