如果你也在 怎样代写凸优化Convex optimization ESE605这个学科遇到相关的难题,请随时右上角联系我们的24/7代写客服。凸优化Convex optimization凸优化是数学优化的一个子领域,研究的是凸集上凸函数最小化的问题。许多类凸优化问题允许采用多项式时间算法,而数学优化一般来说是NP-hard。
凸优化Convex optimization是数学优化的一个子领域,研究的是凸集上凸函数最小化的问题。许多类别的凸优化问题允许采用多项式时间算法,而数学优化一般来说是NP困难的。凸优化在许多学科中都有应用,如自动控制系统、估计和信号处理、通信和网络、电子电路设计、数据分析和建模、金融、统计(最佳实验设计)、和结构优化,其中近似概念被证明是有效的。
凸优化Convex optimization代写,免费提交作业要求, 满意后付款,成绩80\%以下全额退款,安全省心无顾虑。专业硕 博写手团队,所有订单可靠准时,保证 100% 原创。 最高质量的凸优化Convex optimization作业代写,服务覆盖北美、欧洲、澳洲等 国家。 在代写价格方面,考虑到同学们的经济条件,在保障代写质量的前提下,我们为客户提供最合理的价格。 由于作业种类很多,同时其中的大部分作业在字数上都没有具体要求,因此凸优化Convex optimization作业代写的价格不固定。通常在专家查看完作业要求之后会给出报价。作业难度和截止日期对价格也有很大的影响。
海外留学生论文代写;英美Essay代写佼佼者!
EssayTA™有超过2000+名英美本地论文代写导师, 覆盖所有的专业和学科, 每位论文代写导师超过10,000小时的学术Essay代写经验, 并具有Master或PhD以上学位.
EssayTA™在线essay代写、散文、论文代写,3分钟下单,匹配您专业相关写作导师,为您的留学生涯助力!
我们拥有来自全球顶级写手的帮助,我们秉承:责任、能力、时间,为每个留学生提供优质代写服务
论文代写只需三步, 随时查看和管理您的论文进度, 在线与导师直接沟通论文细节, 在线提出修改要求. EssayTA™支持Paypal, Visa Card, Master Card, 虚拟币USDT, 信用卡, 支付宝, 微信支付等所有付款方式.

数学代写|凸优化代写Convex Optimization代考|Testing multiple hypotheses via repeated observations
In the situation of Section 2.5.2.1, given a balanced system of detectors $\phi_{\ell \ell^{\prime}}$ and risks $\epsilon_{\ell \ell^{\prime}}, 1 \leq \ell, \ell^{\prime} \leq L$, for the collection $\mathcal{P}_1, \ldots, \mathcal{P}_L$ (see (2.80)) and a positive integer $K$, we can
pass from detectors $\phi_{\ell \ell^{\prime}}$ and risks $\epsilon_{\ell \ell^{\prime}}$ to the entities
$$
\phi_{\ell \ell^{\prime}}^{(K)}\left(\omega^K=\left(\omega_1, \ldots, \omega_K\right)\right)=\sum_{k=1}^K \phi_{\ell \ell^{\prime}}\left(\omega_k\right), \epsilon_{\ell \ell^{\prime}}^{(K)}=\epsilon_{\ell \ell^{\prime}}^K, 1 \leq \ell, \ell^{\prime} \leq L
$$
associate with the families $\mathcal{P}{\ell}$ families $\mathcal{P}{\ell}^{(K)}$ of probability distributions underlying quasi-stationary $K$-repeated versions of observations $\omega \sim P \in \mathcal{P}{\ell}$-see Section 2.3.2.3 – and thus arrive at hypotheses $H{\ell}^K=\mathcal{H}{\ell}^{\otimes, K}$ stating that the distribution $P^K$ of $K$-repeated observation $\omega^K=\left(\omega_1, \ldots, \omega_K\right), \omega_k \in \Omega$, belongs to the family $\mathcal{P}{\ell}^{\otimes, K}=\bigotimes_{k=1}^K \mathcal{P}{\ell}$, associated with $\mathcal{P}{\ell}$; see Section 2.1.3.3.
By Proposition $2.16$ and (2.80), we arrive at the following analog of (2.80):
$$
\begin{aligned}
&\phi_{\ell \ell^{\prime}}^{(K)}\left(\omega^K\right)+\phi_{\ell^{\prime} \ell}^{(K)}\left(\omega^K\right) \equiv 0, \epsilon_{\ell \ell^{\prime}}^{(K)}=\epsilon_{\ell^{\prime} \ell}^{(K)}=\epsilon_{\ell \ell^{\prime}}^K, \quad 1 \leq \ell, \ell^{\prime} \leq L \
&\forall P^K \in \mathcal{P}{\ell}^{(K)}: \int{\Omega^K} \mathrm{e}^{-\phi_{\ell \ell^{\prime}}^{(K)}\left(\omega^K\right)} P^K\left(d \omega^K\right) \leq \epsilon_{\ell \ell^{\prime}}^{(K)}, \quad 1 \leq \ell, \ell^{\prime} \leq L
\end{aligned}
$$
Given shifts $\alpha_{\ell \ell^{\prime}}$ satisfying (2.82) and applying the construction from Section 2.5.2.2 to these shifts and our newly constructed detectors and risks, we arrive at the test $\mathcal{T}{\mathcal{C}}^K$ deciding on hypotheses $H_1^K, \ldots, H_L^K$ via $K$-repeated observation $\omega^K$. Specifically, given an observation $\omega^K$, the test $\mathcal{T}{\mathcal{C}}^K$ accepts exactly those hypotheses $H_{\ell}^K$ for which $\phi_{\ell \ell^{\prime}}^{(K)}\left(\omega^K\right)-\alpha_{\ell \ell^{\prime}}>0$ whenever $\ell^{\prime}$ is not $\mathcal{C}$-close to $\ell$ :
$$
\mathcal{T}{\mathcal{C}}^K\left(\omega^K\right)=\left{\ell: \phi{\ell \ell^{\prime}}^{(K)}\left(\omega^K\right)-\alpha_{\ell \ell^{\prime}}>0 \forall\left(\ell^{\prime}:\left(\ell, \ell^{\prime}\right) \notin \mathcal{C}\right)\right}
$$
数学代写|凸优化代写Convex Optimization代考|Consistency and near-optimality
Observe that when closeness $\mathcal{C}$ is such that $\epsilon_{\ell \ell^{\prime}}<1$ whenever $\ell, \ell^{\prime}$ are not $\mathcal{C}$-close to each other, the entries on the matrix $\mathcal{E}^{(K)}$ go to 0 as $K \rightarrow \infty$ exponentially fast, whence the $\mathcal{C}$-risk of test $\mathcal{T}{\mathcal{C}}^K$ also goes to 0 as $K \rightarrow \infty$, meaning that test $\mathcal{T}{\mathcal{C}}^K$ is consistent. When, in addition, $\mathcal{P}{\ell}$ correspond to convex hypotheses in a simple o.s., the test $\mathcal{T}{\mathcal{C}}^K$ possesses the property of near-optimality similar to that stated in Proposition 2.25:
Proposition 2.31. Consider the special case of the situation from Section 2.5.2.1 where, given a simple o.s. $\mathcal{O}=\left(\Omega, \Pi ;\left{p_\mu: \mu \in \mathcal{M}\right} ; \mathcal{F}\right)$, the families $\mathcal{P}{\ell}$ of probability distributions are of the form $\mathcal{P}{\ell}=\left{p_\mu: \mu \in N_{\ell}\right}$, where $N_{\ell}, 1 \leq \ell \leq L$, are nonempty convex compact subsets of $\mathcal{M}$. Let also the pairwise detectors $\phi_{\ell \ell^{\prime}}$ and their risks $\epsilon_{\ell \ell^{\prime}}$ underlying the construction from Section 2.5.2.2 be obtained by applying Theorem $2.23$ to the pairs $N_{\ell}, N_{\ell^{\prime}}$, so that for $1 \leq \ell<\ell^{\prime} \leq L$ one has
$$
\phi_{\ell \ell^{\prime}}(\omega)=\frac{1}{2} \ln \left(p_{\mu_{\ell, \ell^{\prime}}}(\omega) / p_{\nu_{\ell, \ell^{\prime}}}(\omega)\right), \epsilon_{\ell \ell^{\prime}}=\exp \left{\operatorname{Opt}{\ell \ell^{\prime}}\right} $$ where $$ \mathrm{Opt}{\ell \ell^{\prime}}=\min {\mu \in N{\ell}, \nu \in N_{\ell^{\prime}}} \ln \left(\int_{\Omega} \sqrt{p_\mu(\omega) p_\nu(\omega)} \Pi(d \omega)\right),
$$
and $\left(\mu_{\ell \ell^{\prime}}, \nu_{\ell \ell^{\prime}}\right)$ form an optimal solution to the optimization problem on the righthand side.
Assume that for some positive integer $K_$ in nature there exists a test $\mathcal{T}^{K_}$ which decides with $\mathcal{C}$-risk $\epsilon \in(0,1 / 2)$, via stationary $K_$-repeated observation $\omega^{K_}$, on the hypotheses $H_{\ell}^{\left(K_\right)}$, stating that the components in $\omega^{K_}$ are drawn, independently of each other, from a distribution $P \in \mathcal{P}{\ell}, \ell=1, \ldots, L$, and let $$ K=\rfloor 2 \frac{1+\ln (L-1) / \ln (1 / \epsilon)}{1-\ln (4(1-\epsilon)) / \ln (1 / \epsilon)} K\lfloor $$ Then the test $\mathcal{T}{\mathcal{C}}^K$ yielded by the construction from Section 2.5.2.2 as applied to the above $\phi{\ell \ell^{\prime}}, \epsilon_{\ell \ell^{\prime}}$, and trivial shifts $\alpha_{\ell \ell^{\prime}} \equiv 0$, decides on the hypotheses $H_{\ell}^K-$ see Section 2.5.2.3-via quasi-stationary $K$-repeated observations $\omega^K$, with $\mathcal{C}$-risk $\leq \epsilon$.
Note that $K / K_ \rightarrow 2$ as $\epsilon \rightarrow+0$.

凸优化代写
数学代写|凸优化代写凸优化代考|通过重复观察检验多个假设
在第2.5.2.1节的情况下,给定一个探测器$\phi_{\ell \ell^{\prime}}$和风险$\epsilon_{\ell \ell^{\prime}}, 1 \leq \ell, \ell^{\prime} \leq L$的平衡系统,对于集合$\mathcal{P}_1, \ldots, \mathcal{P}_L$(见(2.80))和一个正整数$K$,我们可以
从探测器$\phi_{\ell \ell^{\prime}}$和风险$\epsilon_{\ell \ell^{\prime}}$传递到实体
$$
\phi_{\ell \ell^{\prime}}^{(K)}\left(\omega^K=\left(\omega_1, \ldots, \omega_K\right)\right)=\sum_{k=1}^K \phi_{\ell \ell^{\prime}}\left(\omega_k\right), \epsilon_{\ell \ell^{\prime}}^{(K)}=\epsilon_{\ell \ell^{\prime}}^K, 1 \leq \ell, \ell^{\prime} \leq L
$$
与概率分布的族$\mathcal{P}{\ell}$族$\mathcal{P}{\ell}^{(K)}$相关联。$K$ -观测的重复版本$\omega \sim P \in \mathcal{P}{\ell}$ -见2.3.2.3节-从而得到假设$H{\ell}^K=\mathcal{H}{\ell}^{\otimes, K}$,说明$K$ -重复观测$\omega^K=\left(\omega_1, \ldots, \omega_K\right), \omega_k \in \Omega$的分布$P^K$属于家族$\mathcal{P}{\ell}^{\otimes, K}=\bigotimes_{k=1}^K \mathcal{P}{\ell}$,与$\mathcal{P}{\ell}$相关联;参见2.1.3.3节。
通过命题$2.16$和(2.80),我们得到(2.80)的以下类比:
$$
\begin{aligned}
&\phi_{\ell \ell^{\prime}}^{(K)}\left(\omega^K\right)+\phi_{\ell^{\prime} \ell}^{(K)}\left(\omega^K\right) \equiv 0, \epsilon_{\ell \ell^{\prime}}^{(K)}=\epsilon_{\ell^{\prime} \ell}^{(K)}=\epsilon_{\ell \ell^{\prime}}^K, \quad 1 \leq \ell, \ell^{\prime} \leq L \
&\forall P^K \in \mathcal{P}{\ell}^{(K)}: \int{\Omega^K} \mathrm{e}^{-\phi_{\ell \ell^{\prime}}^{(K)}\left(\omega^K\right)} P^K\left(d \omega^K\right) \leq \epsilon_{\ell \ell^{\prime}}^{(K)}, \quad 1 \leq \ell, \ell^{\prime} \leq L
\end{aligned}
$$
给定位移$\alpha_{\ell \ell^{\prime}}$满足(2.82),并将第2.5.2.2节中的构造应用到这些位移和我们新构造的探测器和风险上,我们到达测试$\mathcal{T}{\mathcal{C}}^K$,通过$K$ -重复观察$\omega^K$决定假设$H_1^K, \ldots, H_L^K$。具体地说,给定一个观察结果$\omega^K$,测试$\mathcal{T}{\mathcal{C}}^K$完全接受那些假设$H_{\ell}^K$,其中$\phi_{\ell \ell^{\prime}}^{(K)}\left(\omega^K\right)-\alpha_{\ell \ell^{\prime}}>0$只要$\ell^{\prime}$不是$\mathcal{C}$ -接近$\ell$:
$$
\mathcal{T}{\mathcal{C}}^K\left(\omega^K\right)=\left{\ell: \phi{\ell \ell^{\prime}}^{(K)}\left(\omega^K\right)-\alpha_{\ell \ell^{\prime}}>0 \forall\left(\ell^{\prime}:\left(\ell, \ell^{\prime}\right) \notin \mathcal{C}\right)\right}
$$
数学代写|凸优化代写凸优化代考|一致性和接近最优性
.
观察到当接近度$\mathcal{C}$使$\epsilon_{\ell \ell^{\prime}}<1$当$\ell, \ell^{\prime}$彼此不$\mathcal{C}$时,矩阵$\mathcal{E}^{(K)}$上的条目在$K \rightarrow \infty$指数快速时趋近于0,而测试$\mathcal{T}{\mathcal{C}}^K$的$\mathcal{C}$的风险在$K \rightarrow \infty$时也趋近于0,这意味着测试$\mathcal{T}{\mathcal{C}}^K$一致。此外,当$\mathcal{P}{\ell}$对应一个简单运算系统中的凸假设时,检验$\mathcal{T}{\mathcal{C}}^K$具有类似于命题2.25中所述的近似最优性:
命题2.31。考虑第2.5.2.1节中的特殊情况,其中给定一个简单的os . $\mathcal{O}=\left(\Omega, \Pi ;\left{p_\mu: \mu \in \mathcal{M}\right} ; \mathcal{F}\right)$,概率分布的族$\mathcal{P}{\ell}$是$\mathcal{P}{\ell}=\left{p_\mu: \mu \in N_{\ell}\right}$的形式,其中$N_{\ell}, 1 \leq \ell \leq L$是$\mathcal{M}$的非空凸紧子集。也让在第2.5.2.2节构造的基础上的两两检测器$\phi_{\ell \ell^{\prime}}$及其风险$\epsilon_{\ell \ell^{\prime}}$通过对$N_{\ell}, N_{\ell^{\prime}}$应用定理$2.23$得到,因此对于$1 \leq \ell<\ell^{\prime} \leq L$有
$$
\phi_{\ell \ell^{\prime}}(\omega)=\frac{1}{2} \ln \left(p_{\mu_{\ell, \ell^{\prime}}}(\omega) / p_{\nu_{\ell, \ell^{\prime}}}(\omega)\right), \epsilon_{\ell \ell^{\prime}}=\exp \left{\operatorname{Opt}{\ell \ell^{\prime}}\right} $$,其中$$ \mathrm{Opt}{\ell \ell^{\prime}}=\min {\mu \in N{\ell}, \nu \in N_{\ell^{\prime}}} \ln \left(\int_{\Omega} \sqrt{p_\mu(\omega) p_\nu(\omega)} \Pi(d \omega)\right),
$$
和$\left(\mu_{\ell \ell^{\prime}}, \nu_{\ell \ell^{\prime}}\right)$形成了右边优化问题的最优解
假设对于某个正整数 $K_$ 自然界中存在着一种考验 $\mathcal{T}^{K_}$ 这决定了 $\mathcal{C}$-风险 $\epsilon \in(0,1 / 2)$,通过静止 $K_$重复观测 $\omega^{K_}$,关于假设 $H_{\ell}^{\left(K_\right)}$,说明在 $\omega^{K_}$ 都是从一个分布中独立地画出来的 $P \in \mathcal{P}{\ell}, \ell=1, \ldots, L$,让 $$ K=\rfloor 2 \frac{1+\ln (L-1) / \ln (1 / \epsilon)}{1-\ln (4(1-\epsilon)) / \ln (1 / \epsilon)} K\lfloor $$ 然后是测试 $\mathcal{T}{\mathcal{C}}^K$ 由第2.5.2.2节的结构产生,适用于上述 $\phi{\ell \ell^{\prime}}, \epsilon_{\ell \ell^{\prime}}$,和微不足道的位移 $\alpha_{\ell \ell^{\prime}} \equiv 0$,决定了假设 $H_{\ell}^K-$ 参见2.5.2.3-通过准平稳 $K$-重复观察 $\omega^K$,与 $\mathcal{C}$-风险 $\leq \epsilon$.
注意 $K / K_ \rightarrow 2$ 作为 $\epsilon \rightarrow+0$.
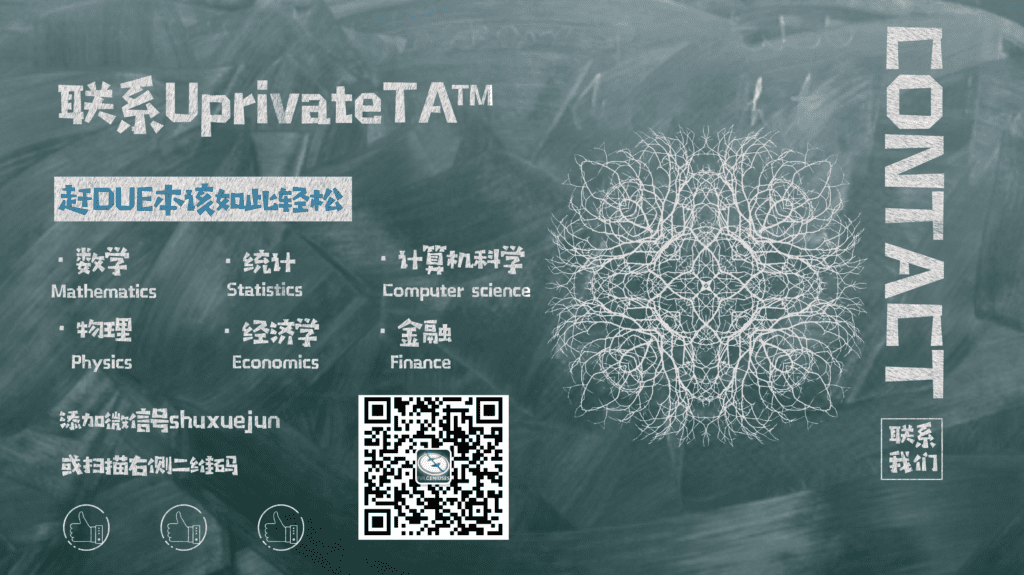
数学代写|凸优化代写Convex Optimization代考 请认准UprivateTA™. UprivateTA™为您的留学生涯保驾护航。
微观经济学代写
微观经济学是主流经济学的一个分支,研究个人和企业在做出有关稀缺资源分配的决策时的行为以及这些个人和企业之间的相互作用。my-assignmentexpert™ 为您的留学生涯保驾护航 在数学Mathematics作业代写方面已经树立了自己的口碑, 保证靠谱, 高质且原创的数学Mathematics代写服务。我们的专家在图论代写Graph Theory代写方面经验极为丰富,各种图论代写Graph Theory相关的作业也就用不着 说。
线性代数代写
线性代数是数学的一个分支,涉及线性方程,如:线性图,如:以及它们在向量空间和通过矩阵的表示。线性代数是几乎所有数学领域的核心。
博弈论代写
现代博弈论始于约翰-冯-诺伊曼(John von Neumann)提出的两人零和博弈中的混合策略均衡的观点及其证明。冯-诺依曼的原始证明使用了关于连续映射到紧凑凸集的布劳威尔定点定理,这成为博弈论和数学经济学的标准方法。在他的论文之后,1944年,他与奥斯卡-莫根斯特恩(Oskar Morgenstern)共同撰写了《游戏和经济行为理论》一书,该书考虑了几个参与者的合作游戏。这本书的第二版提供了预期效用的公理理论,使数理统计学家和经济学家能够处理不确定性下的决策。
微积分代写
微积分,最初被称为无穷小微积分或 “无穷小的微积分”,是对连续变化的数学研究,就像几何学是对形状的研究,而代数是对算术运算的概括研究一样。
它有两个主要分支,微分和积分;微分涉及瞬时变化率和曲线的斜率,而积分涉及数量的累积,以及曲线下或曲线之间的面积。这两个分支通过微积分的基本定理相互联系,它们利用了无限序列和无限级数收敛到一个明确定义的极限的基本概念 。
计量经济学代写
什么是计量经济学?
计量经济学是统计学和数学模型的定量应用,使用数据来发展理论或测试经济学中的现有假设,并根据历史数据预测未来趋势。它对现实世界的数据进行统计试验,然后将结果与被测试的理论进行比较和对比。
根据你是对测试现有理论感兴趣,还是对利用现有数据在这些观察的基础上提出新的假设感兴趣,计量经济学可以细分为两大类:理论和应用。那些经常从事这种实践的人通常被称为计量经济学家。
MATLAB代写
MATLAB 是一种用于技术计算的高性能语言。它将计算、可视化和编程集成在一个易于使用的环境中,其中问题和解决方案以熟悉的数学符号表示。典型用途包括:数学和计算算法开发建模、仿真和原型制作数据分析、探索和可视化科学和工程图形应用程序开发,包括图形用户界面构建MATLAB 是一个交互式系统,其基本数据元素是一个不需要维度的数组。这使您可以解决许多技术计算问题,尤其是那些具有矩阵和向量公式的问题,而只需用 C 或 Fortran 等标量非交互式语言编写程序所需的时间的一小部分。MATLAB 名称代表矩阵实验室。MATLAB 最初的编写目的是提供对由 LINPACK 和 EISPACK 项目开发的矩阵软件的轻松访问,这两个项目共同代表了矩阵计算软件的最新技术。MATLAB 经过多年的发展,得到了许多用户的投入。在大学环境中,它是数学、工程和科学入门和高级课程的标准教学工具。在工业领域,MATLAB 是高效研究、开发和分析的首选工具。MATLAB 具有一系列称为工具箱的特定于应用程序的解决方案。对于大多数 MATLAB 用户来说非常重要,工具箱允许您学习和应用专业技术。工具箱是 MATLAB 函数(M 文件)的综合集合,可扩展 MATLAB 环境以解决特定类别的问题。可用工具箱的领域包括信号处理、控制系统、神经网络、模糊逻辑、小波、仿真等。