如果你也在 怎样代写数据可视化Data VisualizationCS6820这个学科遇到相关的难题,请随时右上角联系我们的24/7代写客服。数据可视化Data Visualization是一个跨学科的领域,处理数据和信息的图形表示。当数据或信息数量众多时,它是一种特别有效的交流方式,例如时间序列。它也是研究抽象数据的视觉表现以加强人类的认知。抽象数据包括数字和非数字数据,如文本和地理信息。它与信息图表和科学可视化有关。
数据可视化Data Visualization是工业界所有应用研究和问题解决中不可缺少的一部分。最基本的数据分析方法是可视化(直方图、散点图、表面图、树状图、平行坐标图等)、统计学(假设检验、回归、PCA等)、数据挖掘(关联挖掘等)和机器学习方法(聚类、分类、决策树等)。在这些方法中,信息可视化,或视觉数据分析,是最依赖人类分析师的认知技能的,并允许发现非结构化的可操作的见解,这些见解只受限于人类的想象力和创造力。分析师不需要学习任何复杂的方法就能够解释数据的可视化。信息可视化也是一种假设生成方案,它可以是,而且通常是在更多的分析或正式分析之后,如统计假设测试。
数据可视化Data Visualization代写,免费提交作业要求, 满意后付款,成绩80\%以下全额退款,安全省心无顾虑。专业硕 博写手团队,所有订单可靠准时,保证 100% 原创。最高质量的数据可视化Data Visualization作业代写,服务覆盖北美、欧洲、澳洲等 国家。 在代写价格方面,考虑到同学们的经济条件,在保障代写质量的前提下,我们为客户提供最合理的价格。 由于作业种类很多,同时其中的大部分作业在字数上都没有具体要求,因此数据可视化Data Visualization作业代写的价格不固定。通常在专家查看完作业要求之后会给出报价。作业难度和截止日期对价格也有很大的影响。
海外留学生论文代写;英美Essay代写佼佼者!
EssayTA™有超过2000+名英美本地论文代写导师, 覆盖所有的专业和学科, 每位论文代写导师超过10,000小时的学术Essay代写经验, 并具有Master或PhD以上学位.
EssayTA™在线essay代写、散文、论文代写,3分钟下单,匹配您专业相关写作导师,为您的留学生涯助力!
我们拥有来自全球顶级写手的帮助,我们秉承:责任、能力、时间,为每个留学生提供优质代写服务
论文代写只需三步, 随时查看和管理您的论文进度, 在线与导师直接沟通论文细节, 在线提出修改要求. EssayTA™支持Paypal, Visa Card, Master Card, 虚拟币USDT, 信用卡, 支付宝, 微信支付等所有付款方式.

数据科学代写|数据可视化代写Data Visualization代考|Uncertainty in Sample Statistics
In the first two sections of this chapter, we presented methods for visualizing the distribution of values for one or more variables. In this section, we discuss the visualization of the variability that results from statistical sampling. The process of using sample data to make estimates of or draw conclusions about one or more characteristics of a population is called statistical inference.
A common example of statistical inference is political polling. Consider an example in which members of a political party in Texas are considering the support of a particular candidate for election to the U.S. Senate, and party leaders want to estimate the proportion of registered voters in the state that favor the candidate. Suppose a sample of 400 registered voters in Texas is selected, and 160 of those voters indicate a preference for the candidate. Thus, an estimate of proportion of the population of registered voters who favor the candidate is $160 / 400=0.40$. However, because this sample of 400 is only a portion of the voter population of Texas, some error or deviation is to be expected between the sample proportion and the population proportion that we are estimating. That is, there is uncertainty in how close the sample proportion is to the population proportion.
Another example of statistical inference arises in market research. Consider the situation where a sample of weekly grocery bills is collected to estimate the average amount of money spent on groceries by a target population of potential customers of a grocery delivery service. Suppose a sample of 100 weekly grocery bills is selected, and the sample mean is $\$ 102.70$. However, because this sample of 100 is only a portion of possible weekly grocery bills by potential customers, some error or deviation between the sample mean and the population mean that we are estimating is to be expected. That is, there is uncertainty in how close the sample mean is to the population mean.
In this section, we will discuss how to portray the uncertainty in sample-based estimates of proportions and means. Failure to appropriately convey the inherent uncertainty in these estimates may lead the audience to develop a false sense of confidence in these point estimates. We want to construct visualizations that help the audience comprehend these sample-based estimates as intervals rather than points.
数据科学代写|数据可视化代写Data Visualization代考|Displaying a Confidence Interval on a Mean
Because the sample mean cannot be expected to provide the exact value of the population mean, a confidence interval is computed by adding and subtracting a value, called the margin of error, to the sample mean:
sample mean $\pm$ margin of error
The purpose of a confidence interval is to provide information about how close the sample mean may be to the value of the population mean. While the derivation of the formula for the margin of error for a confidence interval on a mean is beyond the scope of this book, we note that it is dependent on three factors: (1) the sample size, (2) how variable the sample values are (as measured by the sample standard deviation), and (3) with how much confidence we want to claim that the population mean lies within the interval. As the sample size increases, the margin of error decreases. This is intuitive because as we collect more data, we should be able to better estimate the mean. As the sample standard deviation increases, the margin of error increases. This is intuitive because as the values in the data demonstrate more variation, it becomes more difficult to estimate the mean. Finally, as the required confidence level increases, the margin of error increases. If we must state an interval with more confidence, then we must be more conservative with that interval and increase its width. Common levels of confidence are $95 \%$ and $99 \%$.
Using the file DeathAvgAgeChart, we demonstrate the calculations of the margin of error for a $95 \%$ confidence interval on a mean. In Figure 5.24, we compute the sample mean, the sample standard deviation, and sample size for the female and male observations in the cell range E2:F4 using the appropriate Excel functions. In cells E5 and F5, we compute the margin of error on the mean for the female and male samples, respectively, using the CONFIDENCE.T function. The CONFIDENCE.T formula requires three input arguments, =CONFIDENCE.T(significance level, std dev, sample size). For a 95\% confidence interval, the first argument of the CONFIDENCE.T function is $1-0.95=0.05$, and is called the level of significance. The second and third arguments of the CONFIDENCE.T function are the sample standard deviation and the sample size, respectively.

数据可视化代写
数据科学代写|数据可视化代写Data Visualization代考|Uncertainty in Sample Statistics
在本章的前两节中,我们介绍了可视化一个或多个变量的值分布的方法。在本节中,我们将讨论统计抽样产生的可变性的可视化。使用样本数据对总体的一个或多个特征进行估计或得出结论的过程称为统计推断。
统计推断的一个常见例子是政治投票。考虑一个例子,德克萨斯州的一个政党成员正在考虑支持特定候选人竞选美国参议院,而政党领导人想要估计该州登记选民中支持该候选人的比例。假设选择了德克萨斯州 400 名登记选民的样本,其中 160 名选民表示对候选人的偏好。因此,对支持该候选人的登记选民人口比例的估计为160/400=0.40. 但是,由于这 400 个样本只是德克萨斯州选民人口的一部分,因此预计样本比例与我们正在估计的人口比例之间会出现一些误差或偏差。也就是说,样本比例与总体比例的接近程度存在不确定性。
统计推断的另一个例子出现在市场研究中。考虑收集每周杂货账单样本以估计杂货配送服务的潜在客户的目标人群在杂货上花费的平均金额的情况。假设选择了 100 份每周杂货账单的样本,样本均值为$102.70. 但是,由于这 100 个样本只是潜在客户每周可能的杂货账单的一部分,因此我们估计的样本均值与总体均值之间存在一些误差或偏差是可以预料的。也就是说,样本均值与总体均值的接近程度存在不确定性。
在本节中,我们将讨论如何描述基于样本的比例和均值估计中的不确定性。未能恰当地传达这些估计中固有的不确定性可能会导致听众对这些点估计产生错误的信心。我们希望构建可视化,帮助观众将这些基于样本的估计理解为间隔而不是点。
数据科学代写|数据可视化代写Data Visualization代考|Displaying a Confidence Interval on a Mean
因为不能期望样本均值提供总体均值的准确值,所以通过在样本均值上加上和减去一个称为误差边际的值来计算置信区间:
样本均值±误差范围
置信区间的目的是提供有关样本均值与总体均值的接近程度的信息。虽然均值置信区间的误差范围公式的推导超出了本书的范围,但我们注意到它取决于三个因素:(1)样本量,(2)样本的可变性值是(通过样本标准差测量),以及(3)我们想要声称总体平均值位于区间内的置信度有多大。随着样本量的增加,误差幅度减小。这是直观的,因为随着我们收集更多数据,我们应该能够更好地估计平均值。随着样本标准差的增加,误差幅度增加。这是直观的,因为随着数据中的值显示出更多的变化,估计平均值变得更加困难。最后,随着所需置信水平的增加,误差幅度也会增加。如果我们必须更自信地陈述一个区间,那么我们必须对该区间更加保守并增加其宽度。常见的置信水平是95%和99%.
使用文件 DeathAvgAgeChart,我们演示了计算95%均值的置信区间。在图 5.24 中,我们使用适当的 Excel 函数计算单元格范围 E2:F4 中女性和男性观察值的样本均值、样本标准差和样本大小。在单元格 E5 和 F5 中,我们使用 CONFIDENCE.T 函数分别计算女性和男性样本的均值误差范围。CONFIDENCE.T 公式需要三个输入参数,=CONFIDENCE.T(significance level, std dev, sample size)。对于 95% 置信区间,CONFIDENCE.T 函数的第一个参数是1−0.95=0.05, 称为显着性水平。CONFIDENCE.T 函数的第二个和第三个参数分别是样本标准差和样本大小。
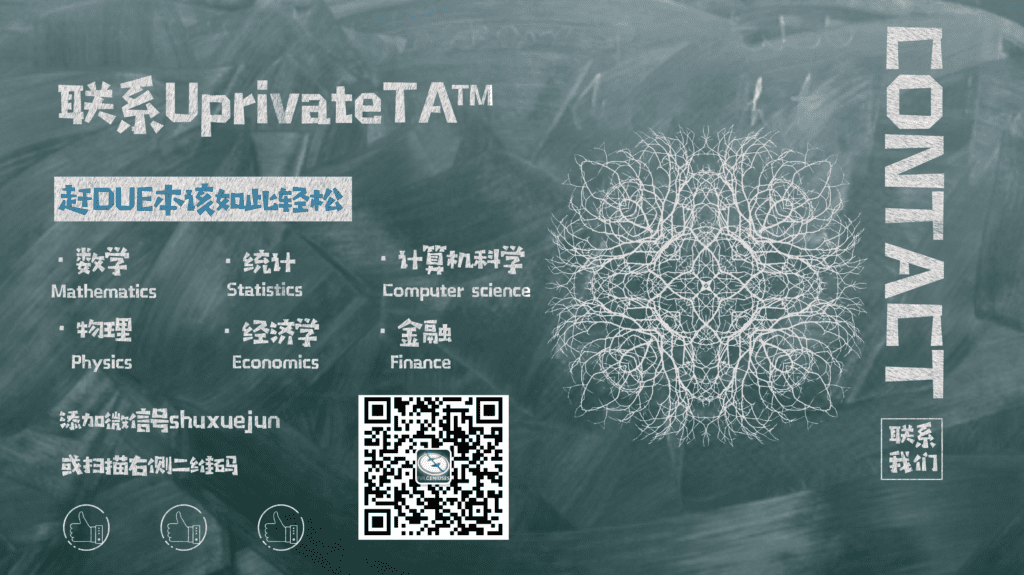
数据科学代写|数据可视化代写Data Visualization代考 请认准UprivateTA™. UprivateTA™为您的留学生涯保驾护航。
微观经济学代写
微观经济学是主流经济学的一个分支,研究个人和企业在做出有关稀缺资源分配的决策时的行为以及这些个人和企业之间的相互作用。my-assignmentexpert™ 为您的留学生涯保驾护航 在数学Mathematics作业代写方面已经树立了自己的口碑, 保证靠谱, 高质且原创的数学Mathematics代写服务。我们的专家在图论代写Graph Theory代写方面经验极为丰富,各种图论代写Graph Theory相关的作业也就用不着 说。
线性代数代写
线性代数是数学的一个分支,涉及线性方程,如:线性图,如:以及它们在向量空间和通过矩阵的表示。线性代数是几乎所有数学领域的核心。
博弈论代写
现代博弈论始于约翰-冯-诺伊曼(John von Neumann)提出的两人零和博弈中的混合策略均衡的观点及其证明。冯-诺依曼的原始证明使用了关于连续映射到紧凑凸集的布劳威尔定点定理,这成为博弈论和数学经济学的标准方法。在他的论文之后,1944年,他与奥斯卡-莫根斯特恩(Oskar Morgenstern)共同撰写了《游戏和经济行为理论》一书,该书考虑了几个参与者的合作游戏。这本书的第二版提供了预期效用的公理理论,使数理统计学家和经济学家能够处理不确定性下的决策。
微积分代写
微积分,最初被称为无穷小微积分或 “无穷小的微积分”,是对连续变化的数学研究,就像几何学是对形状的研究,而代数是对算术运算的概括研究一样。
它有两个主要分支,微分和积分;微分涉及瞬时变化率和曲线的斜率,而积分涉及数量的累积,以及曲线下或曲线之间的面积。这两个分支通过微积分的基本定理相互联系,它们利用了无限序列和无限级数收敛到一个明确定义的极限的基本概念 。
计量经济学代写
什么是计量经济学?
计量经济学是统计学和数学模型的定量应用,使用数据来发展理论或测试经济学中的现有假设,并根据历史数据预测未来趋势。它对现实世界的数据进行统计试验,然后将结果与被测试的理论进行比较和对比。
根据你是对测试现有理论感兴趣,还是对利用现有数据在这些观察的基础上提出新的假设感兴趣,计量经济学可以细分为两大类:理论和应用。那些经常从事这种实践的人通常被称为计量经济学家。
MATLAB代写
MATLAB 是一种用于技术计算的高性能语言。它将计算、可视化和编程集成在一个易于使用的环境中,其中问题和解决方案以熟悉的数学符号表示。典型用途包括:数学和计算算法开发建模、仿真和原型制作数据分析、探索和可视化科学和工程图形应用程序开发,包括图形用户界面构建MATLAB 是一个交互式系统,其基本数据元素是一个不需要维度的数组。这使您可以解决许多技术计算问题,尤其是那些具有矩阵和向量公式的问题,而只需用 C 或 Fortran 等标量非交互式语言编写程序所需的时间的一小部分。MATLAB 名称代表矩阵实验室。MATLAB 最初的编写目的是提供对由 LINPACK 和 EISPACK 项目开发的矩阵软件的轻松访问,这两个项目共同代表了矩阵计算软件的最新技术。MATLAB 经过多年的发展,得到了许多用户的投入。在大学环境中,它是数学、工程和科学入门和高级课程的标准教学工具。在工业领域,MATLAB 是高效研究、开发和分析的首选工具。MATLAB 具有一系列称为工具箱的特定于应用程序的解决方案。对于大多数 MATLAB 用户来说非常重要,工具箱允许您学习和应用专业技术。工具箱是 MATLAB 函数(M 文件)的综合集合,可扩展 MATLAB 环境以解决特定类别的问题。可用工具箱的领域包括信号处理、控制系统、神经网络、模糊逻辑、小波、仿真等。