如果你也在 怎样代写抽样调查Survey sampling STA4222这个学科遇到相关的难题,请随时右上角联系我们的24/7代写客服。抽样调查Survey sampling在美国,对家庭人口进行概率抽样的常见方法是地区概率抽样、随机数字拨号电话抽样,以及最近的基于地址的抽样。在概率抽样中,有一些专门的技术,如分层抽样和聚类抽样,在不改变概率抽样的基本原则的情况下,提高了抽样过程的精度或效率。
抽样调查Survey sampling分层是指在抽样前,根据每个样本单位的辅助信息,将人口成员划分为同质的子组的过程。分层应该是相互排斥的:人口中的每个元素都必须被分配到一个分层中。分层也应该是集体详尽的:不能排除任何人口元素。然后,在每个层中可以采用简单随机抽样或系统抽样等方法。分层通常通过减少抽样误差来提高样本的代表性。
essayta.™抽样调查Survey sampling代写,免费提交作业要求, 满意后付款,成绩80\%以下全额退款,安全省心无顾虑。专业硕 博写手团队,所有订单可靠准时,保证 100% 原创。essayta.™, 最高质量的抽样调查Survey sampling作业代写,服务覆盖北美、欧洲、澳洲等 国家。 在代写价格方面,考虑到同学们的经济条件,在保障代写质量的前提下,我们为客户提供最合理的价格。 由于统计Statistics作业种类很多,同时其中的大部分作业在字数上都没有具体要求,因此抽样调查Survey sampling作业代写的价格不固定。通常在经济学专家查看完作业要求之后会给出报价。作业难度和截止日期对价格也有很大的影响。
想知道您作业确定的价格吗? 免费下单以相关学科的专家能了解具体的要求之后在1-3个小时就提出价格。专家的 报价比上列的价格能便宜好几倍。
essayta.™ 为您的留学生涯保驾护航 在统计代写方面已经树立了自己的口碑, 保证靠谱, 高质且原创的统计代考服务。我们的专家在抽样调查Survey sampling代写方面经验极为丰富,各种抽样调查Survey sampling相关的作业也就用不着 说。
我们提供的抽样调查Survey sampling STA4222及其相关学科的代写,服务范围广, 其中包括但不限于:

统计代写|抽样调查代考SURVEY SAMPLING代考|Linearization Technique
Suppose $\theta_{1}, \cdots, \theta_{K}$ are $K(>1)$ finite population parameters admitting unbiased estimators $t_{1}, \cdots, t_{K}$ respectively based on a sample $s$ chosen according to a sampling design $p$. Let us require that a non-linear function $\phi\left(\theta_{1}, \cdots, \theta_{K}\right)$ of these parameters be suitably estimated. For example, let $\theta_{1}=N, \theta_{2}=\sum_{1}^{N} y_{i}, \theta_{3}=\sum_{1}^{N} x_{i}, \theta_{4}=\sum_{1}^{N} y_{i}^{2}, \theta_{5}=\sum_{1}^{N} x_{i}^{2}$ and $\theta_{6}=\sum_{1}^{N} x_{i} y_{i}$ and our intention be to suitably estimate
$$
\begin{aligned}
R &=\frac{N \sum_{1}^{N} x_{i} y_{i}-\sum_{1}^{N} x_{i} \sum y_{i}}{\sqrt{N \sum x_{i}^{2}-\left(\sum x_{i}\right)^{2}} \sqrt{N \sum_{1}^{N} y_{i}^{2}-\left(\sum_{1}^{N} y_{i}\right)^{2}}} \
&=\frac{\theta_{1} \theta_{6}-\theta_{2} \theta_{3}}{\sqrt{\theta_{1} \theta_{5}-\theta_{3}^{2}} \sqrt{\theta_{1} \theta_{4}-\theta_{2}^{2}}}
\end{aligned}
$$
which is the finite population correlation between $x$ and $y$.
In general, let $\theta=\left(\theta_{1}, \cdots, \theta_{K}\right), t=\left(t_{1}, \cdots, t_{K}\right)$. Expanding $\phi(t)$ around $\theta$ by Taylor’s series expansion we may write
$$
\phi(t)=\phi(\theta)+\sum_{j=1}^{K} \frac{\partial \phi(\theta)}{\partial \theta_{j}}\left(t_{j}-\theta_{j}\right)
$$
neglecting the higher order terms supposing $\left(t_{j}-\theta_{j}\right)$ ‘s are numerically small, assuming sample-size to be large enough.
Let $\theta_{j}=\sum_{i=1}^{N} \xi_{j i}, t_{j}=\sum_{1}^{N} \xi_{j i} b_{s j i} I_{s i}$ with $b_{s j i}$ ‘s free of elements $\xi_{j i}, i=$ $1 \cdots N$
$$
\begin{aligned}
&\lambda_{j}=\frac{\partial}{\partial \theta_{j}} \phi(\theta), j=1, \cdots, K \text { and } \
&\sum_{j=1}^{K} \lambda_{j} t_{j}=\sum_{1}^{N} \psi_{i} b_{s i} I_{s i}, \psi_{i}=\sum_{j=1}^{K} \lambda_{j} \xi_{j i}
\end{aligned}
$$
so,
$E \phi(t) \simeq \phi(\theta)$ and
$$
V \phi(t) \simeq V\left[\sum_{1}^{N} \psi_{i} b_{s i} I_{s i}\right]
$$
$$
=\sum_{i=1}^{N} \psi_{i}^{2} C_{i}+\sum_{i \neq j}^{N} \sum_{i \neq j}^{N} \psi_{i} \psi_{j} C_{i j}
$$
writing
$$
\begin{aligned}
C_{i} &=\sum_{s \ni i} b_{s i}^{2} p(s)-1 \text { and } \
C_{i j} &=\sum_{s \ni i, j} b_{s i} b_{s j} p(s)-1
\end{aligned}
$$
统计代写|抽样调查代考SURVEY SAMPLING代考|Small Area Estimation
On this topic J.N.K. Rao (2003), J.N.K. Rao and Isabel Molina (2015), and Mukhopadhyay (1998) have three book-level expansions, and in addition, the present author (Chaudhuri, 2012) has an electronic monograph. In the latter’s three textbooks ${($ Chaudhuri and Stenger (2005), Chaudhuri $(2010$, 2014) $}$ also, certain aspects of this topic have been dealt with.
Let me offer here some of our ideas about this. Suppose a sample has been chosen from a survey population following a suitable sampling design $p$. Let such a sample $s$ chosen with probability $p(s)$ be surveyed chiefly to appropriately estimate the population total and mean by some standard procedures. Let further the population be composed of certain discernible non-overlapping parts called ‘domains’ labelled $U_{d}$ with $s \cap U_{d}=s_{d}$ for $d=1, \cdots, D$ and $\cup_{d=1}^{D} U_{d}=U$. Let, in addition, it be needed to suitably estimate the totals and means for $U_{d}$ ‘s, $d=1, \cdots, D$ utilizing the sample data $\left(s, y_{i} \mid i \in s\right)$ at hand. Suppose the size of $s$ is $n$ which has been suitably hit upon to provide adequate efficiency for the population total/mean estimate. But the size $n_{d}$ of $s_{d}$ is a random variable for $d=1, \cdots, D$. If the estimator for the population total $Y=\sum_{i=1}^{N} y_{i}$ is taken as the Horvitz and Thompson’s estimator $t_{H T}=\sum_{i \in s} \frac{y_{i}}{\pi_{i}}$ and is supposedly adequate enough in efficiency one has to decide on a serviceable estimator for the $d$ th domain total $Y_{d}=\sum_{i \in U_{d}} y_{i}$ as well.
Let us write
$$
\begin{aligned}
I_{d i} &=1 \text { if } i \in U_{d} \
&=0 \text { if } i \notin U_{d} \
Y_{d} &=\sum_{i=1}^{N} y_{i} I_{d i}=\sum_{i \in U_{d}} y_{d i}, y_{d i}=y_{i} I_{d i}
\end{aligned}
$$
and $N_{d}$ as the size of $U_{d} ;$ also $\bar{Y}{d}=\frac{Y{d}}{N_{d}}$, the $d$ th domain mean, $d=1 \cdots, D$.

抽样调查代写
统计代写|抽样调查代考SURVEY SAMPLING代考|Linearization Technique
认为 $\theta_{1}, \cdots, \theta_{K}$ 是 $K(>1)$ 允许无偏估计里的有限总体参数 $t_{1}, \cdots, t_{K}$ 分别基于一个样本 $s$ 根据抽样设 计选择 $p$. 让我们要求一个非线性函数 $\phi\left(\theta_{1}, \cdots, \theta_{K}\right)$ 这些参数的适当估计。例如, 让
$\theta_{1}=N, \theta_{2}=\sum_{1}^{N} y_{i}, \theta_{3}=\sum_{1}^{N} x_{i}, \theta_{4}=\sum_{1}^{N} y_{i}^{2}, \theta_{5}=\sum_{1}^{N} x_{i}^{2}$ 和 $\theta_{6}=\sum_{1}^{N} x_{i} y_{i}$ 我们的意图是 适当地估计
$$
R=\frac{N \sum_{1}^{N} x_{i} y_{i}-\sum_{1}^{N} x_{i} \sum y_{i}}{\sqrt{N \sum x_{i}^{2}-\left(\sum x_{i}\right)^{2}} \sqrt{N \sum_{1}^{N} y_{i}^{2}-\left(\sum_{1}^{N} y_{i}\right)^{2}}} \quad=\frac{\theta_{1} \theta_{6}-\theta_{2} \theta_{3}}{\sqrt{\theta_{1} \theta_{5}-\theta_{3}^{2}} \sqrt{\theta_{1} \theta_{4}-\theta_{2}^{2}}}
$$
这是之间的有限总体相关性 $x$ 和 $y$.
一般来说, 令 $\$ \mathrm{~ 垚 t 月 ⿱}$
$\backslash$ phi $(I)=\backslash$ phi $(\perp$ theta $)+\backslash$ sum ${j=1}^{\wedge}{K} \backslash$ frac ${\backslash$ partial $\backslash$ phi $(\perp$ theta $)} \backslash \backslash$ partial
$\backslash \mathrm{~ t h e t a _ { j } } \ l e f t (}$
$\$ \$$
忽略高阶项假设 $\left(t_{j}-\theta_{j}\right)$ 的数值很小, 假设样本里足够大。
$\begin{aligned}&\text { 让 } \theta_{j} \&\$ \$\end{aligned} \sum_{i=1}^{N} \xi_{j i}, t_{j}=\sum_{1}^{N} \xi_{j i} b_{s j i} I_{s i}$ 和 $b_{s j i}$ 没有元素 $\xi_{j i}, i=1 \cdots N$
$\$ begin{aligned $}$
$\& \backslash$ lambda_{j}=\frac ${\backslash$ partial $} \backslash \backslash$ partial \theta_{j}} \phi( $\backslash$ theta $),$
$\& \backslash$ sum_{j=1 $}^{\wedge}{\mathrm{K}} \backslash$ lambda_{j} ${ }{-}{\mathrm{j}}=\backslash$ sum{1 $}^{\wedge}{\mathrm{N}} \backslash$ psi_{i} b_{si $}$ I_$_{-}{\mathrm{si}}, \backslash$ psi_{i}
$=\backslash$ sum_{j=1$}^{\wedge}{\mathrm{K}} \backslash$ lambda_{j} \xi_{ji}
\end{aligned }
$\$ \$$
所以,
$\$ \mathrm{E} \backslash$ phi $(\pm) \backslash$ simeq $\backslash$ phi $(\backslash$ theta $)$ and $\$$
$\vee \backslash$ phi $(\pm) \backslash$ simeq $\vee \backslash$ left[ $\backslash \backslash$ sum_{_ ${1} \wedge{N} \backslash$ psi_{i} b_{si $}$ I_{si} $\backslash$ right $]$
writing
$C_{i}=\sum_{s \ni i} b_{s i}^{2} p(s)-1$ and $C_{i j}=\sum_{s \ni i, j} b_{s i} b_{s j} p(s)-1$
$\$ \$$
统计代写|抽样调查代考SURVEY SAMPLING代考|Small Area Estimation
在这个主题上, JNKRao (2003)、JNK Rao 和 Isabel Molina (2015) 以及 Mukhopadhyay (1998) 有三个书耤级别的扩展, 此外, 目前的作者 (Chaudhuri, 2012) 有一本电子专着。在后者的三本教科书 中(\$ChaudhuriandStenger $(2005)$, Chaudhuri\$ $(2010 \$, 2014)$ \$此外, 还讨论了该主题的某些 方面。
让我在这里提出我们对此的一些想法。假设已按照适当的抽样设计丛调查总体中选择样本 $p$. 让这样一个 样本 $s$ 以概率选择 $p(s)$ 调查主要是为了通过一些标准程序适当地估计人口总数和平均值。让总体由某些 可识别的非重焏部分组成, 称为 “域”, 标记为 $U_{d}$ 和 $s \cap U_{d}=s_{d}$ 为 此外, 我们需要适当地估计总数和手段 $U_{d}$ 的, $d=1, \cdots, D$ 利用样本数据 $\left(s, y_{i} \mid i \in s\right)$ 在眼前。假 设大小为 $s$ 是 $n$ 它已被适当地触及, 以为人口总数/平均估计提供足够的效率。但是尺寸 $n_{d}$ 的 $s_{d}$ 是一个随 机变里 $d=1, \cdots, D$. 如果人口总数的估计里 $Y=\sum_{i=1}^{N} y_{i}$ 被视为 Horvitz 和 Thompson 的估计里 $t_{H T}=\sum_{i \in s} \frac{y_{i}}{\pi_{i}}$ 并且据说在效率上已经足够了, 因此必须为 $d$ 总域名 $Y_{d}=\sum_{i \in U_{d}} y_{i}$ 也是。
让我们写
$$
I_{d i}=1 \text { if } i \in U_{d} \quad=0 \text { if } i \notin U_{d} Y_{d}=\sum_{i=1}^{N} y_{i} I_{d i}=\sum_{i \in U_{d}} y_{d i}, y_{d i}=y_{i} I_{d i}
$$
和 $N_{d}$ 作为大小 $U_{d} ;$ 还有 $\$ \backslash$ bar {}${d}=\backslash$ frac ${Y{\mathrm{~d}}}\left{\mathrm{N}_{-}{\mathrm{d}}\right}$, the $\mathrm{d}$ thdomainmean, $\mathrm{d}=1 \backslash \mathrm{cdots}, \mathrm{D} \$$ 。
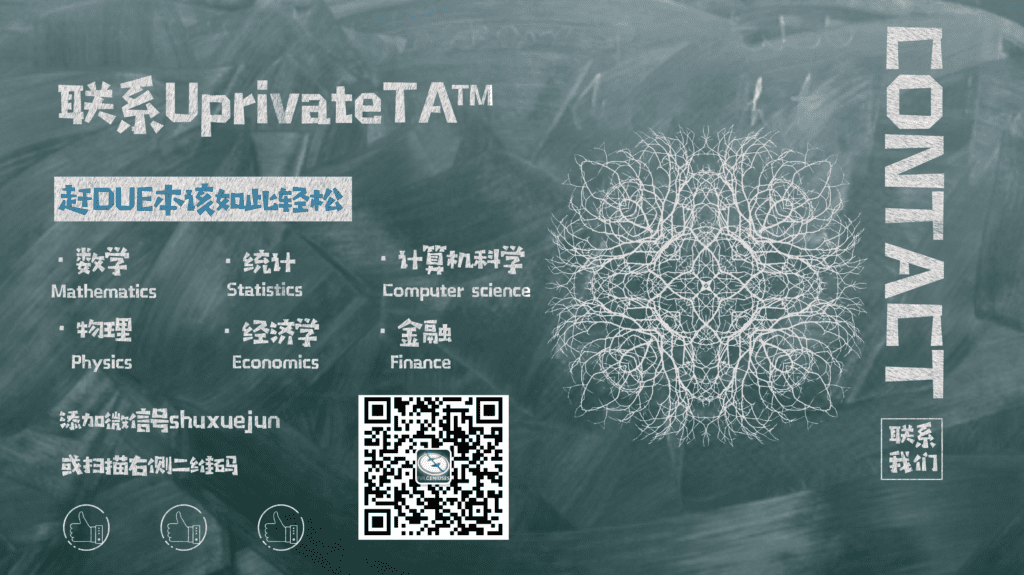
统计代写|抽样调查代考Survey sampling代考 请认准UprivateTA™. UprivateTA™为您的留学生涯保驾护航。
微观经济学代写
微观经济学是主流经济学的一个分支,研究个人和企业在做出有关稀缺资源分配的决策时的行为以及这些个人和企业之间的相互作用。my-assignmentexpert™ 为您的留学生涯保驾护航 在数学Mathematics作业代写方面已经树立了自己的口碑, 保证靠谱, 高质且原创的数学Mathematics代写服务。我们的专家在图论代写Graph Theory代写方面经验极为丰富,各种图论代写Graph Theory相关的作业也就用不着 说。
线性代数代写
线性代数是数学的一个分支,涉及线性方程,如:线性图,如:以及它们在向量空间和通过矩阵的表示。线性代数是几乎所有数学领域的核心。
博弈论代写
现代博弈论始于约翰-冯-诺伊曼(John von Neumann)提出的两人零和博弈中的混合策略均衡的观点及其证明。冯-诺依曼的原始证明使用了关于连续映射到紧凑凸集的布劳威尔定点定理,这成为博弈论和数学经济学的标准方法。在他的论文之后,1944年,他与奥斯卡-莫根斯特恩(Oskar Morgenstern)共同撰写了《游戏和经济行为理论》一书,该书考虑了几个参与者的合作游戏。这本书的第二版提供了预期效用的公理理论,使数理统计学家和经济学家能够处理不确定性下的决策。
微积分代写
微积分,最初被称为无穷小微积分或 “无穷小的微积分”,是对连续变化的数学研究,就像几何学是对形状的研究,而代数是对算术运算的概括研究一样。
它有两个主要分支,微分和积分;微分涉及瞬时变化率和曲线的斜率,而积分涉及数量的累积,以及曲线下或曲线之间的面积。这两个分支通过微积分的基本定理相互联系,它们利用了无限序列和无限级数收敛到一个明确定义的极限的基本概念 。
计量经济学代写
什么是计量经济学?
计量经济学是统计学和数学模型的定量应用,使用数据来发展理论或测试经济学中的现有假设,并根据历史数据预测未来趋势。它对现实世界的数据进行统计试验,然后将结果与被测试的理论进行比较和对比。
根据你是对测试现有理论感兴趣,还是对利用现有数据在这些观察的基础上提出新的假设感兴趣,计量经济学可以细分为两大类:理论和应用。那些经常从事这种实践的人通常被称为计量经济学家。
MATLAB代写
MATLAB 是一种用于技术计算的高性能语言。它将计算、可视化和编程集成在一个易于使用的环境中,其中问题和解决方案以熟悉的数学符号表示。典型用途包括:数学和计算算法开发建模、仿真和原型制作数据分析、探索和可视化科学和工程图形应用程序开发,包括图形用户界面构建MATLAB 是一个交互式系统,其基本数据元素是一个不需要维度的数组。这使您可以解决许多技术计算问题,尤其是那些具有矩阵和向量公式的问题,而只需用 C 或 Fortran 等标量非交互式语言编写程序所需的时间的一小部分。MATLAB 名称代表矩阵实验室。MATLAB 最初的编写目的是提供对由 LINPACK 和 EISPACK 项目开发的矩阵软件的轻松访问,这两个项目共同代表了矩阵计算软件的最新技术。MATLAB 经过多年的发展,得到了许多用户的投入。在大学环境中,它是数学、工程和科学入门和高级课程的标准教学工具。在工业领域,MATLAB 是高效研究、开发和分析的首选工具。MATLAB 具有一系列称为工具箱的特定于应用程序的解决方案。对于大多数 MATLAB 用户来说非常重要,工具箱允许您学习和应用专业技术。工具箱是 MATLAB 函数(M 文件)的综合集合,可扩展 MATLAB 环境以解决特定类别的问题。可用工具箱的领域包括信号处理、控制系统、神经网络、模糊逻辑、小波、仿真等。