如果你也在 怎样代写金融计量经济学Financial Econometrics MTH9891这个学科遇到相关的难题,请随时右上角联系我们的24/7代写客服。金融计量经济学Financial Econometrics发表金融计量经济学研究的优质期刊包括《计量经济学》、《计量经济学杂志》和《商业与经济统计杂志》。金融计量学杂志》专门研究金融计量经济学。它由Federico Bandi和Andrew Patton编辑,并与SoFiE有密切的关系。
金融计量经济学Financial Econometrics与其他形式的计量经济学不同,因为其重点通常是分析在竞争性、流动性市场上交易的金融资产的价格。在金融业工作或研究金融部门的人经常在一系列活动中使用计量经济学技术–例如,在支持投资组合管理和证券估值方面。金融计量学对风险管理至关重要,因为了解在未来几天、几周、几个月和几年内 “坏 “的投资结果预计会发生多少次是很重要的。
essayta.com™金融计量经济学Financial Econometrics代写,免费提交作业要求, 满意后付款,成绩80\%以下全额退款,安全省心无顾虑。专业硕 博写手团队,所有订单可靠准时,保证 100% 原创。essayta.com™, 最高质量的金融计量经济学Financial Econometrics作业代写,服务覆盖北美、欧洲、澳洲等 国家。 在代写价格方面,考虑到同学们的经济条件,在保障代写质量的前提下,我们为客户提供最合理的价格。 由于统计Statistics作业种类很多,同时其中的大部分作业在字数上都没有具体要求,因此金融计量经济学Financial Econometrics作业代写的价格不固定。通常在经济学专家查看完作业要求之后会给出报价。作业难度和截止日期对价格也有很大的影响。
想知道您作业确定的价格吗? 免费下单以相关学科的专家能了解具体的要求之后在1-3个小时就提出价格。专家的 报价比上列的价格能便宜好几倍。
essayta.com™ 为您的留学生涯保驾护航 在网课代修方面已经树立了自己的口碑, 保证靠谱, 高质且原创的网课代考服务。我们的专家在金融计量经济学Financial Econometrics代写方面经验极为丰富,各种金融计量经济学Financial Econometrics相关的作业也就用不着 说。
我们提供的金融计量经济学Financial Econometrics MTH9891及其相关学科的代写,服务范围广, 其中包括但不限于:

金融代写|金融计量经济学代考FINANCIAL ECONOMETRICS代考|Regression 1, σ2 Known
We use here the beloved cars dataset from $\mathrm{R}$, which measured the speed of 50 cars (in $\mathrm{mph}$ ) and how long it took them to stop (in feet). Speeds ranged from 4 to 25 $\mathrm{mph}$, and distances from 2 to 120 feet. In order for $\sigma$ to be known, we cheated, and used the estimate from the ordinary linear regression of speed on distance. Figure 3 shows the results.
Now this data set has been used innumerable times to illustrate regression techniques, but I believe it is the first time it has been demonstrated how truly awful regression is here.
In each panel, the predictive posterior is given in black, and the parameter posterior is given in dashed red. In order to highlight the comparisons, the parameter posterior was shifted to the peak of the predictive posterior distributions. The parameter posterior is of course fixed-and at the “effect” size for speed. Here it has a mean of $3.9$, with credible interval of $(3.1,4.8)$.
It is immediately clear just reporting the parameter posterior implies vastly more certainty than the predictive posteriors. We do not have just one predictive posterior, but one for every possible level of speed. Hence we also have varying levels of overcertainty. The ratio of predictive to parameter credible intervals was, $40.1$ (at $1 \mathrm{mph}$ ), $37.8(10 \mathrm{mph}), 37.6(20 \mathrm{mph})$, and $40.1$ (30 $\mathrm{mph})$,
The over-certainty is immense at any speed. But what is even more interesting is the enormous probability leakage at low speeds. Here we have most probability for predictive stopping distances of less than 0 , a physical impossibility. The background information B did not account for this impossibility, and merely said, as most do say, that a regression is a fine approximation. It is not. It stinks for low speeds.
But this astonishingly failure of the model would have gone forever unnoticed had the model not been cast in its predictive form. The parameter-centric analysis would be have missed, and almost always does miss, this glaring error.
If instead of $\sigma^{2}$ being known, and faked, we let it be unknown, the results for this model are much the same, only with greater uncertainties in the posteriors. We skip that and move to a more general example.
金融代写|金融计量经济学代考FINANCIAL ECONOMETRICS代考|Regression 2, σ2 Unknown
It is well to take an example which in most respects passes muster, even in a predictive sense, but which nevertheless still exaggerates certainty. We use the oats data in the MASS package. This was an experiment with three oat varieties planted at 6 blocks, and with four amounts of added nitrogen via manure: none added, $0.2 \mathrm{cwt}, 0.4 \mathrm{cwt}$, and $0.6$ cwt per acre. The outcome is yield of oats in quarter pounds. The intent and supposition was that greater amounts of nitrogen would lead to greater yields.
The first step is computing an ordinary linear regression; here using Gibbs sampling via the rstanarm package version $2.18 .2$ in $\mathrm{R}$ version 3.5.2, using default priors, but here using 6 chains of 20,000 iterations each for greater resolution. Diagnostics (not shown) suggest the model converged. The results are in Fig. $4 .$
The predictive posteriors are presented as histograms, using posterior observable draws, and the parameter posteriors as red dashed lines. We chose the first block and the Golden rain oat variety to create the predictions. There is some difference between blocks, but little between varieties. A full analysis would, of course, consider all these arrangements, but here these choices will be sufficient. The parameter posteriors are cut off as before so as not to lose resolution on the observables. The parameter posteriors are also re-centered for easy visual comparison by adding the mean intercept value.
The over-certainty as measured by length of the predictive to parameteric credible intervals is about 3 times for each amount of nitrogen. However, since the purpose of this was to demonstrate increasing nitrogen boosts yields, this measure may seem out of place. Rather, the mistake of assuming the uncertainties are the same is not likely to be made.
There is still lurking over-certainty, though.
The posterior probability the parameter for $0.2 \mathrm{cwt}$ added nitrogen is greater than 0 is very close to $1(0.9998)$. Any researcher would go away with the idea that adding nitrogen guarantees boosting yield. But the posterior predictive chance that a new plot with $0.2$ cwt will have a greater yield than a plot with no added nitrogen is only $0.8$. This implies an over-certainty of $\approx 1 / 0.8=1.25$.
Likewise, although all the parameters in the model for nitrogen have extremely high probabilities of differing from each other, the predictive probabilities of greater yields do not differ as much. The posterior probability the parameter for $0.4 \mathrm{cwt}$ is greater than $0.2$ cwt is $0.997$, and the parameter for $0.6$ cwt is greater than $0.4$ cwt is $0.96$.
The predictive probability of greater yields in plots with $0.4 \mathrm{cwt}$ over $0.2 \mathrm{cwt}$ is $0.75$, with over-certainty of $0.997 / 0.75=1.33$. And the predictive probability of greater yields in plots with $0.6$ cwt over $0.4$ cwt is $0.66$, with over-certainty of $0.96 / 0.66=1.45$.
Notice that we were able to compute over-certainty in this case because we were comparing probabilities for “like” things, here oats yields. We cannot always make comparisons, however, as the last section details.

金融计量经济学代写
金融代写|金融计量经济学代考FINANCIAL ECONOMETRICS代考|Regression 1, σ2 Known
我们在这里使用心爱的汽车数据集 $R$, 它测量了 50 辆汽车的速度 (在 $\mathrm{mph}$ ) 以及他们停下来花了多长时间 (以英尺为单位)。速度范围从 4 到 $25 \mathrm{mph}$, 距离为 2 到 120 英尺。为了 $\sigma$ 众所周知, 我们作贲, 并使用 了速度对距离的普通线性回归的估计。图 3 显示了结果。
现在这个数据集已经被无数次用来说明回归技术, 但我相信这是第一次证明回归是多么可怕。
在每个面板中, 预测后验以黑色给出, 参数后验以红色虚线给出。为了突出比较, 㕕数后验被转移到预测后 验分布的峰值。参数后验当然是固定的, 并且是速度的“影响”大小。这里的意思是 $3.9$, 可信区间为 $(3.1,4.8)$.
很明显, 仅报告参数后验比预测后验意味着更多的确定性。我们不仅有一个预测后验, 而且每个可能的速度 水平都有一个。因此, 我们也有不同程度的过度确定性。预测与参数可信区间的比率为, $40.1$ (在 $1 \mathrm{mph}$ ), $37.8(10 \mathrm{mph}), 37.6(20 \mathrm{mph})$, 和 $40.1(30 \mathrm{mph})$,
过度确定性在任何速度下都是巨大的。但更有诹的是低速时的巨大概率泄漏。在这里, 我们最有可能预测停 止距离小于 0 , 这是物理上的不可能。背景信息 $B$ 没有说明这种可能性, 只是说, 正如大多数人所说, 回 归是一个很好的近似值。它不是。低速时会发真。
但是, 如果该模型不是以预测形式进行的, 那么该模型的这种惊人的失败将永远被忽视。以参数为中心的分 析会错过, 而且几平总是会错过这个明显的错误。
如果不是 $\sigma^{2}$ 已知和伪造, 我们让它不为人知, 这个模型的结果大致相同, 只是后验的不确定性更大。我们跳 过它并转到更一般的示例。
金融代写|金融计量经济学代考FINANCIAL ECONOMETRICS代考|Regression 2, σ2 Unknown
举一个在大多数方面都合格的例子是很好的, 即使是在预测意义上, 但仍然夸大了确定性。我们使用 MASS 包中的燕麦数据。这是一个实验, 在 6 个街区种植了三个燕麦品种, 并通过肥料添加了四种鲤的氮: 不添 加, $0.2 \mathrm{cwt}, 0.4 \mathrm{cwt}$, 和 $0.6$ 英担每英亩。结果是以四分之一磅为单位的燕麦产荲。意图和假设是, 更多 的矛会导致更高的产量。
第一步是计算一个普通的线性回归; 这里通过 rstanarm 包版本使用 Gibbs 采样 2.18.2在 $\mathrm{R}$ 版本 3.5.2, 使 用默认先验, 但这里使用 6 个链, 每个涟 20,000 次迭代以获得更高的分辨率。诊断 (末显示) 表明模型收 敛。结果如图。 4 .
预测后验以直方图的形式呈现, 使用后验可观察图, 参数后验以红色虚线表示。我们选择了第一个区块和金 雨燕麦品种来创建预测。块之间有一些差异, 但品种之间的差异很小。当然, 全面分析会考虑所有这些安 排, 但在这里这些选择就足够了。参数后验像以前一样被切断, 以免失去对可观察量的分辨率。参数后验也 重新居中, 以便通过泘加平均截距值进行视觉比较。
通过预测到参数可信区间的长度测量的过度确定性对于每种氮量约为 3 倍。然而, 由于这样做的目的是为了 证明增加氮肥的产量, 这一措施可能看起来不合适。相反, 假设不确定性相同的错误不太可能发生。
不过, 仍然㳻伏着过度的确定性。
参数的后验概率 $0.2 \mathrm{cwt}$ 加氮大于 0 非常接近 $1(0.9998)$. 任何研究人员都会放弃添加氮可以提高产量的想 法。但是一个新的情节的后验预测机会 $0.2 \mathrm{cwt}$ 将比没有㴁加氮的地块具有更高的产量 $0.8$. 这意味着过度确 定 $\approx 1 / 0.8=1.25$.
同样, 尽管氮模型中的所有参数都具有极高的相互差异概率, 但更高产量的预测概率差异不大。参数的后验 概率 $0.4 \mathrm{cwt}$ 大 $0.2 \mathrm{cwt}$ 是 $0.997$, 和参数 $0.6 \mathrm{cwt}$ 大于 $0.4 \mathrm{cwt}$ 是 $0.96$.
地块中更高产量的预测概率 $0.4 \mathrm{cwt}$ 超过 $0.2 \mathrm{cwt}$ 是 $0.75$, 具有过确定性 $0.997 / 0.75=1.33$. 以及在具有更 高产量的地块中的预测概率 $0.6$ 英担超过 $0.4 \mathrm{cwt}$ 是 $0.66$, 具有过确定性 $0.96 / 0.66=1.45$.
请注意, 在这种情况下, 我们能够计算过度确定性, 因为我们正在比较“相似”事物的概率, 这里是燕麦产 鲤。然而, 正如最后一节所详述的, 我们不能总是进行比较。
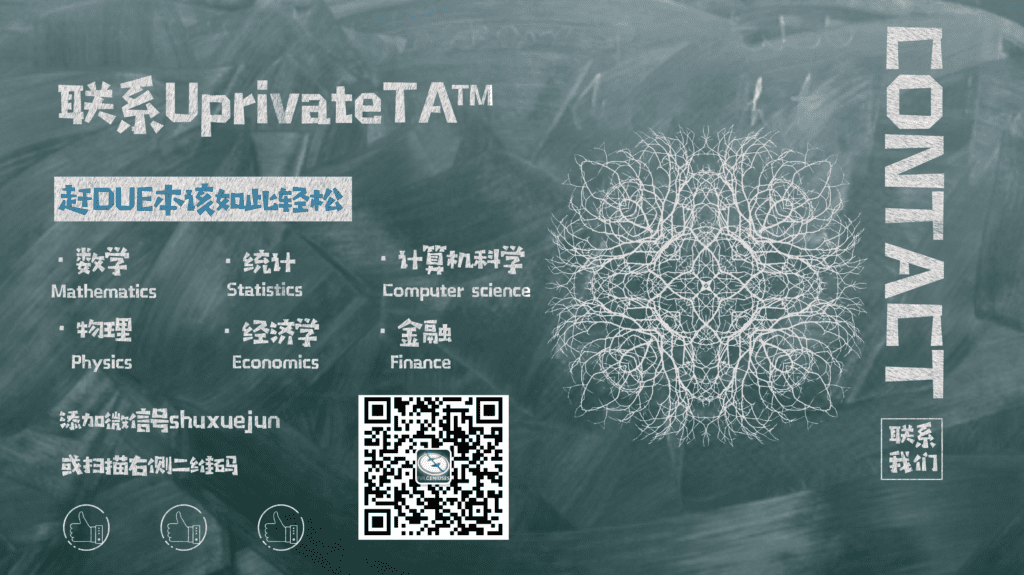
金融代写|金融计量经济学代考Financial Econometrics代考 请认准UprivateTA™. UprivateTA™为您的留学生涯保驾护航。
微观经济学代写
微观经济学是主流经济学的一个分支,研究个人和企业在做出有关稀缺资源分配的决策时的行为以及这些个人和企业之间的相互作用。my-assignmentexpert™ 为您的留学生涯保驾护航 在数学Mathematics作业代写方面已经树立了自己的口碑, 保证靠谱, 高质且原创的数学Mathematics代写服务。我们的专家在图论代写Graph Theory代写方面经验极为丰富,各种图论代写Graph Theory相关的作业也就用不着 说。
线性代数代写
线性代数是数学的一个分支,涉及线性方程,如:线性图,如:以及它们在向量空间和通过矩阵的表示。线性代数是几乎所有数学领域的核心。
博弈论代写
现代博弈论始于约翰-冯-诺伊曼(John von Neumann)提出的两人零和博弈中的混合策略均衡的观点及其证明。冯-诺依曼的原始证明使用了关于连续映射到紧凑凸集的布劳威尔定点定理,这成为博弈论和数学经济学的标准方法。在他的论文之后,1944年,他与奥斯卡-莫根斯特恩(Oskar Morgenstern)共同撰写了《游戏和经济行为理论》一书,该书考虑了几个参与者的合作游戏。这本书的第二版提供了预期效用的公理理论,使数理统计学家和经济学家能够处理不确定性下的决策。
微积分代写
微积分,最初被称为无穷小微积分或 “无穷小的微积分”,是对连续变化的数学研究,就像几何学是对形状的研究,而代数是对算术运算的概括研究一样。
它有两个主要分支,微分和积分;微分涉及瞬时变化率和曲线的斜率,而积分涉及数量的累积,以及曲线下或曲线之间的面积。这两个分支通过微积分的基本定理相互联系,它们利用了无限序列和无限级数收敛到一个明确定义的极限的基本概念 。
计量经济学代写
什么是计量经济学?
计量经济学是统计学和数学模型的定量应用,使用数据来发展理论或测试经济学中的现有假设,并根据历史数据预测未来趋势。它对现实世界的数据进行统计试验,然后将结果与被测试的理论进行比较和对比。
根据你是对测试现有理论感兴趣,还是对利用现有数据在这些观察的基础上提出新的假设感兴趣,计量经济学可以细分为两大类:理论和应用。那些经常从事这种实践的人通常被称为计量经济学家。
MATLAB代写
MATLAB 是一种用于技术计算的高性能语言。它将计算、可视化和编程集成在一个易于使用的环境中,其中问题和解决方案以熟悉的数学符号表示。典型用途包括:数学和计算算法开发建模、仿真和原型制作数据分析、探索和可视化科学和工程图形应用程序开发,包括图形用户界面构建MATLAB 是一个交互式系统,其基本数据元素是一个不需要维度的数组。这使您可以解决许多技术计算问题,尤其是那些具有矩阵和向量公式的问题,而只需用 C 或 Fortran 等标量非交互式语言编写程序所需的时间的一小部分。MATLAB 名称代表矩阵实验室。MATLAB 最初的编写目的是提供对由 LINPACK 和 EISPACK 项目开发的矩阵软件的轻松访问,这两个项目共同代表了矩阵计算软件的最新技术。MATLAB 经过多年的发展,得到了许多用户的投入。在大学环境中,它是数学、工程和科学入门和高级课程的标准教学工具。在工业领域,MATLAB 是高效研究、开发和分析的首选工具。MATLAB 具有一系列称为工具箱的特定于应用程序的解决方案。对于大多数 MATLAB 用户来说非常重要,工具箱允许您学习和应用专业技术。工具箱是 MATLAB 函数(M 文件)的综合集合,可扩展 MATLAB 环境以解决特定类别的问题。可用工具箱的领域包括信号处理、控制系统、神经网络、模糊逻辑、小波、仿真等。